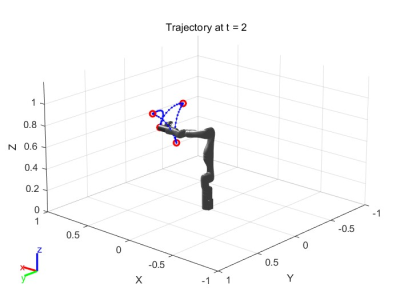
SSADMO algorithm is proposed to study the optimal spatial trajectories of sea buckthorn fruit vibration separation. In this study, kinematics interpolation analysis of manipulator trajectories was carried out to compare the trajectories of cubic polynomials and quintic polynomial interpolation, and to compare the trajectories and attitudes of the manipulators under the condition of limited operating range and speed, in this paper, we propose a new SSADMO algorithm which combines the advantages of SSA and DMO optimization algorithms in the 3-5-3 polynomial interpolation.
- Categories: