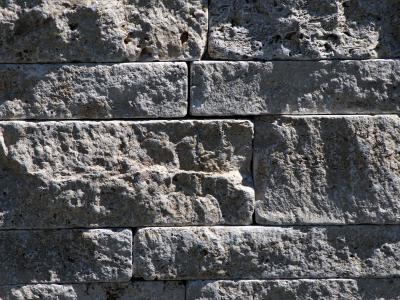
Smart contract performance
- Categories:
Smart contract performance
This paper introduces an aircraft engine remaining life prediction model based on an improved Transformer architecture (SBi-Transformer), addressing the computational inefficiencies and inadequate local temporal dependency capturing capabilities of the standard transformer model in processing long sequence data. The SBi-Transformer employs a dual-layer attention mechanism to reduce computational complexity and enhance local temporal dependencies.
<p class="MsoNormal"><span style="mso-spacerun: 'yes'; font-family: 宋体; mso-ascii-font-family: Calibri; mso-hansi-font-family: Calibri; mso-bidi-font-family: 'Times New Roman'; font-size: 10.5000pt; mso-font-kerning: 1.0000pt;"><span style="font-family: Calibri;">This dataset contains expert evaluations of various text features using Grey Relational Analysis (GRA), comparing the performance of original and new prompt words.
Large Vision-Language Models (LVLMs) struggle with distractions, particularly in the presence of irrelevant visual or textual inputs. This paper introduces the Irrelevance Robust Visual Question Answering (IR-VQA) benchmark to systematically evaluate and mitigate this ``multimodal distractibility". IR-VQA targets three key paradigms: irrelevant visual contexts in image-independent questions, irrelevant textual contexts in image-dependent questions, and text-only distractions.
Decompilation, the process of translating machine-level code into human-readable source code, is critical in reverse engineering. While its main purpose is to facilitate code comprehension when the original source code is unavailable, the understandability of decompiled code is important. However, existing research has mainly focused on the correctness of decompilation, with limited attention given to the decompilation understandability.
This dataset provides a GraphML‐based representation of an interdependent infrastructure system, covering power, water, transportation, and community infrastructures. Each node is annotated with key attributes such as resource type, criticality, societal vulnerability score (SVS), and capacity—while directed edges capture the flow or dependency relationships among these resources. The dataset facilitates resilience and recovery analysis by enabling repair order simulation, based on certain metrics‐based prioritization.
The TripAdvisor online airline review dataset, spanning from 2016 to 2023, provides a comprehensive collection of passenger feedback on airline services during the COVID-19 pandemic. This dataset includes user-generated reviews that capture sentiments, preferences, and concerns, allowing for an in-depth analysis of shifting customer priorities in response to pandemic-related disruptions. By examining these reviews, the dataset facilitates the study of evolving passenger expectations, changes in service perceptions, and the airline industry's adaptive strategies.
This is a videoconference between a witness about murders who is a victim of many crimes and a law firm. This witness is called Colin Paul Gloster. This law firm is called Pais do Amaral Advogados.
\begin{quotation}``A significant part of the background of eh this process is that Hospital
Sobral Cid tortured me during 2013 eh but eh this process is actually
about consequences eh thru through a later process [. . .] To obscure eh
this torture of 2013, eh a new show trial was intitiated. Its process was
This study investigates the worst-case Single-Event Effect (SEE) response in semiconductor
power devices induced by energetic ions. An existing predictive model is refined and extended to potentially
consider various semiconductor materials, other geometries and technologies. Comprehensive expressions
for predicting critical ion energies that lead to worst-case SEE response in semiconductor power devices are
obtained through computational simulations. A dedicated experiment is conducted to investigate the model
This dataset pertains to degradation testing utilizing tightened threshold censoring. It comprises various forms of data, including simulation data that provide insights into theoretical models and expected outcomes. Additionally, it includes test result data, which document the actual performance and behavior of the products under testing conditions. The repeatability test data are also part of this dataset, which ensures that the results are consistent and reproducible across multiple tests.