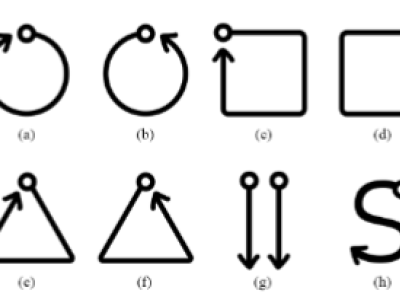
This dataset comprises high-resolution 3-axis accelerometer recordings collected from human participants performing distinct hand gestures, intended for training gesture-based assistive interfaces. Each participant’s raw motion signals are individually organized, enabling both user-specific and generalizable model development. The dataset includes time-series accelerometer data, along with a feature-augmented version containing extracted statistical and temporal descriptors such as RMS, Jerk, Entropy, and SMA.
- Categories: