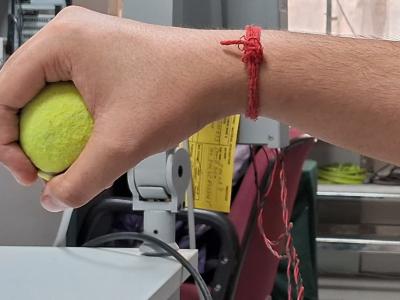
This study aims to create a robust hand grasp recognition system using surface electromyography (sEMG) data collected from four electrodes. The grasps to be utilized in this study include cylindrical grasp, spherical grasp, tripod grasp, lateral grasp, hook grasp, and pinch grasp. The proposed system seeks to address common challenges, such as electrode shift, inter-day difference, and individual difference, which have historically hindered the practicality and accuracy of sEMG-based systems.
- Categories: