occlusion-induced corrupted radar echo dataset, constructed for low-altitude UAV monitoring scenarios
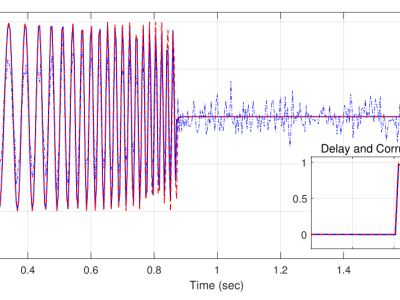
- Citation Author(s):
-
Shuai Xu (Pengcheng Labtory)
- Submitted by:
- Shuai Xu
- Last updated:
- DOI:
- 10.21227/qw6h-ns61
- Data Format:
- Categories:
- Keywords:
Abstract
In low-altitude unmanned aerial vehicle (UAV) detection scenarios, the initial segment of radar linear frequency modulation (LFM) signals is often corrupted due to building occlusions and noise interference, making accurate range estimation difficult. To address this issue, we propose a deep learning-based framework named Deep Time-Frequency Inverse Reconstruction Network (DTFIRNet) for radar echo signal restoration and precise ranging. DTFIRNet integrates an enhanced UNet architecture with a deep inverse short-time Fourier transform (iSTFT) module, effectively leveraging the time–frequency representation (TFR) provided by the STFT to guide waveform reconstruction. Specifically, residual connections and spatio-temporal attention mechanisms are incorporated to significantly improve the feature representation capability of UNet under complex occlusion conditions, enabling high-quality TFR reconstruction and waveform restoration. Moreover, we introduce, for the first time, a trainable deep iSTFT module to achieve efficient transformation from the frequency domain to the time domain. Experimental results demonstrate that DTFIRNet outperforms existing state-of-the-art methods in terms of TFR reconstruction quality, waveform recovery accuracy, and final ranging error, reducing the average ranging error to 0.611 \textit{m}. In addition, a novel dataset featuring LFM radar signals with occlusion and noise corruption is constructed and publicly released via \textsc{IEEEDataPort}, providing new theoretical and data support for low-altitude UAV monitoring and radar signal processing research.
Instructions:
see "intro_dataport.pdf"