PD_dataset
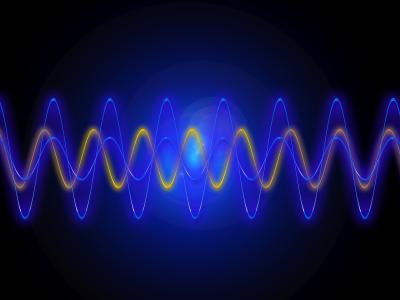
- Citation Author(s):
- Submitted by:
- Francis Oraekwe
- Last updated:
- DOI:
- 10.21227/0tz7-jt13
- Data Format:
- Categories:
- Keywords:
Abstract
The development of automated techniques for speech analysis-based Parkinson's disease (PD) detection has attracted a lot of interest, especially because of its possible uses in health tele-monitoring. Due to the drawbacks of the ᾳ - Synuclein Seed Amplification Assay technique, scientists are looking more closely at speech signals as a potential substitute for PD detection. In order to identify PD, this proposal describes a thorough investigation that emphasizes using both voice and unvoiced source material. Acquiring relative speech data is part of the methodology, which is followed by pitch synchronous and block processing for data preprocessing. Cloud computing will also be used for computational requirements and data storage. Feature extraction will be made easier by the Enhanced Simple Inverse Filter Tracking (ESIFT) technique, and classification will be carried out by combining Support Vector Machines, XGBoost, and LightGBM methods. Finally, to attain the best possible solution for a voice recognition system, use the Ensemble technique of supervised machine learning with the goal of a detection accuracy of more than 80%. When compared to current speech detection systems, the expected result is a notable increase in accuracy.
Instructions:
This dataset, which was finished on November 5, 2018, is for sale in the field of speech analysis and Parkinson's disease research training. The study's data were from 758 people, both men and women, with PD and in good health, and their ages ranged from 33 to 87. This dataset is only meant for study in the area of speech processing of parkinson's disease. it contains both normal and abnormal speech signals, all labeled.