Ni-HGr: A sEMG dataset for hand grasp recognition in non-ideal conditions
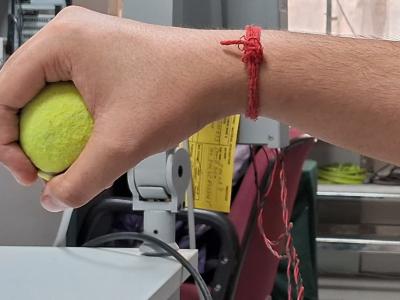
- Citation Author(s):
- Submitted by:
- Rishi Sharma
- Last updated:
- DOI:
- 10.21227/wmvg-hb17
- Data Format:
- Links:
- Categories:
- Keywords:
Abstract
This study aims to create a robust hand grasp recognition system using surface electromyography (sEMG) data collected from four electrodes. The grasps to be utilized in this study include cylindrical grasp, spherical grasp, tripod grasp, lateral grasp, hook grasp, and pinch grasp. The proposed system seeks to address common challenges, such as electrode shift, inter-day difference, and individual difference, which have historically hindered the practicality and accuracy of sEMG-based systems. By addressing these issues, the researchers intend to develop a more reliable and user-friendly interface for applications in prosthetic devices, rehabilitation systems, and human-computer interaction. The challenge of electrode shift refers to the slight movement or repositioning of electrodes on the skin, which can occur due to natural muscle contractions or external factors, leading to variations in the sEMG signals. Similarly, inter-day differences arise from variations across different recording days. Also, individual difference which demonstrate variability between individuals through factors like body mass, thickness of surface muscles, etc. These factors contribute to inconsistencies in the sEMG signals, making it challenging to achieve stable and repeatable gesture recognition. Furthermore, creating a system that is independent of specific electrode locations on the arm enhances the device's adaptability and ease of use, allowing for greater flexibility in real-world applications. This project will employ advanced signal processing and machine learning techniques to mitigate the effects of these variables, thereby ensuring high accuracy and reliability in hand grasp recognition regardless of the electrode position, day of recording or individual difference. The ultimate goal is to develop a versatile sEMG-based control system that can be seamlessly integrated into various practical applications, enhancing the quality of life for users.
Instructions:
Ni-HGr DATASET
This repository contains components utilized in the research study entitled "Ni-HGr: Non-Ideal Analysis for sEMG-Based Hand Grasp Recognition using Multi-Band Mutual Information". The article has been submitted for possible publication with IEEE Transactions on Human-Machine Systems.
Overview
This study aims to create a robust hand grasp recognition system using surface electromyography (sEMG) data collected from four electrodes. The grasps to be utilized in this study include cylindrical grasp, spherical grasp, tripod grasp, lateral grasp, hook grasp, and pinch grasp. The proposed system seeks to address common challenges, such as electrode shift, inter-day difference, and individual difference, which have historically hindered the practicality and accuracy of sEMG-based systems. By addressing these issues, the researchers intend to develop a more reliable and user-friendly interface for applications in prosthetic devices, rehabilitation systems, and human-computer interaction. The challenge of electrode shift refers to the slight movement or repositioning of electrodes on the skin, which can occur due to natural muscle contractions or external factors, leading to variations in the sEMG signals. Similarly, inter-day differences arise from variations across different recording days. Also, individual difference which demonstrate variability between individuals through factors like body mass, thickness of surface muscles, etc. These factors contribute to inconsistencies in the sEMG signals, making it challenging to achieve stable and repeatable gesture recognition. Furthermore, creating a system that is independent of specific electrode locations on the arm enhances the device's adaptability and ease of use, allowing for greater flexibility in real-world applications. This project will employ advanced signal processing and machine learning techniques to mitigate the effects of these variables, thereby ensuring high accuracy and reliability in hand grasp recognition regardless of the electrode position, day of recording or individual difference. The ultimate goal is to develop a versatile sEMG-based control system that can be seamlessly integrated into various practical applications, enhancing the quality of life for users.
Dataset details
This study is approved by the Office of Defence Institute of Advanced Technology, Pune, India. The dataset was collected at the Sensors and Signal Intelligence (SenSigI) Lab, Department of Electronics Engineering at the Defence Institute of Advanced Technology in Pune, India. The experimental setup consists of Salus 4C EMG machine (i.e., 4 channel EMG machine) and a fixed size bracelet of circumference 23 cm and diameter of 7.32 cm for angular estimation. The sEMG electrodes are connected on an elbow support band to maintain their initial alignment. Six intact-abled participants (2 females and 4 males) between the ages 20 and 27 years participated in the EMG data collection process in non-ideal conditions. These include electrode shift, individual difference, and inter-day difference. During the experimental session, each participant was seated in a chair with their hands in a resting position. EMG data was collected over 5 different days. On each day, 6 participants executed 5 experiments at 5 different angular positions (i.e., 0°, 5°, 10°, 355°, 350°). At every position, participants were instructed to perform 6 hand grasp movements, including cylindrical, spherical, tripod, lateral, hook, and pinch grasps. Each grasp type consisted of 5 trials. Each grasp was executed for a duration of 4-5 seconds, followed by an inter-grasp interval of 6-7 seconds. The inter-grasp interval was divided into an approximate 2-second return phase, a 2-second rest phase, and a 2-second reach phase.
Data arrangement
The data collected from each day is stored in different cells in .mat format. The data for each day is formatted and saved as: emg_data{1,v}{1,o}{1,c}{1,m}{1,n}.mat, where v represents number of days (i.e., v = 1,2,...,5), o indicate number of subjects (i.e., o = 1,2,...,6), c defines the angular positions which are arranged in order (i.e., c = 0°, 10°, 350°, 355°, and 5° ), m represents six hand grasp movements in order of cylindrical (CY), hook (HO), lateral (LA), pinch (PI), spherical (SP), and tripod (TR) grasps, and n indicate number of trials.
Note: One trial of SP grasp from 0° angular position of subject 6 from day 4 is excluded from the dataset.
Reference:
Sharma, S. and Sharma, R. R., Ni-HGr: Non-Ideal Analysis for sEMG-Based Hand Grasp Recognition using Multi-Band Mutual Information. (Submitted in IEEE Transactions on Human-Machine Systems)