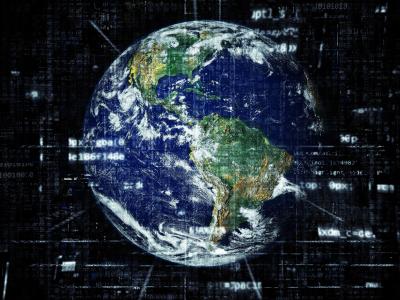
Device identification using network traffic analysis is being researched for IoT and non-IoT devices against cyber-attacks. The idea is to define a device specific unique fingerprint by analyzing the solely inter-arrival time (IAT) of packets as feature to identify a device. Deep learning is used on IAT signature for device fingerprinting of 58 non-IoT devices. We observed maximum recall and accuracy of 97.9% and 97.7% to identify device. A comparitive research GTID found using defined IAT signature that models of device identification are better than device type identification.
- Categories: