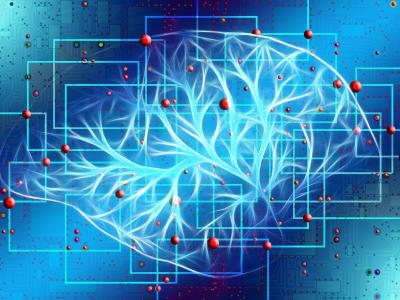
Unmanned aerial vehicles (UAVs) are being used for various applications, but the associated cyber risks are also increasing. Machine learning techniques have been successfully adopted to develop intrusion detection systems (IDSs). However, none of the existing works published the cyber or physical datasets that have been used to develop the IDS, which hinders further research in this field.
- Categories: