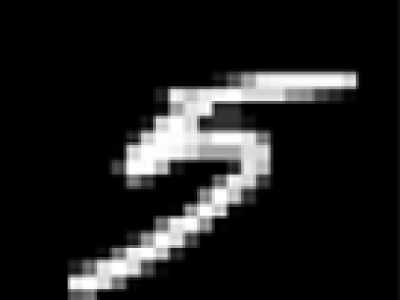
The rapid advancement of deep neural network (DNN) models has enabled their widespread application across various domains, including face recognition and natural language processing. However, data-driven DNN models are prone to erroneous behavior when inadequately trained, necessitating extensive predictive labeling of test data to identify and mitigate defects. Manual labeling, however, remains both labor-intensive and inefficient.
- Categories: