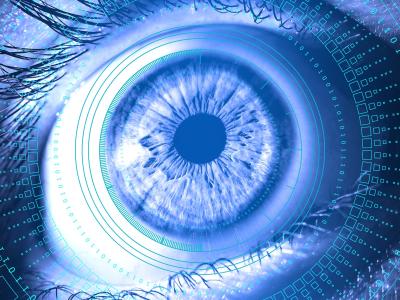
The dataset includes eight urban scenes of different sizes and styles, as well as various lighting and weather conditions. Each scene contains 200 vehicles of different types, 100 pedestrians and 5,000 RGB images, semantic images, and point cloud files. The annotations include both the depth and 2D information of the objects.
- Categories: