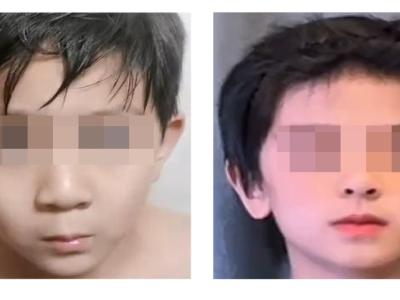
This paper introduces the Chinese Social Media Autism Children Dataset (CSMACD), a novel resource for autism spectrum disorder (ASD) research. CSMACD compiles high-definition, unobstructed frontal facial images of Chinese children (aged 6 months to 15 years) with ASD, sourced from mainstream social media platforms (e.g., Bilibili, Douyin, and Tencent Video). Videos were identified using ASD-related keywords (e.g., "autism," "Star Baby") and recommendation algorithms.
- Categories: