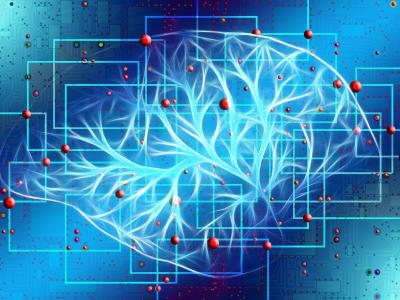
This is a preprocessed dataset of 2 companies from Pakistan Stock Exchange.
- Categories:
This is a preprocessed dataset of 2 companies from Pakistan Stock Exchange.
In this paper, we present a collaborative recommend system that recommends elective courses for students based on similarities of student’s grades obtained in the last semester. The proposed system employs data mining techniques to discover patterns between grades. Consequently, we have noticed that clustering students into similar groups by performing clustering. The data set is processed for clustering in such a way that it produces optimal number of clusters.
The data contains files which are unprocessed and signals which pass through alert signal separator.
This dataset is offered as .csv and is part of 3 files which are:
- File 1: has all 1699 arabic news headlines colllected with the corresponding emotion classification that 3 annotators agreed on with no bias
- File 2: has the dataset with BOW features extracted
- File 3: has the dataset with n-gram features extracted
The supplementary files of our submitted TIFS paper: "CALPA-NET: Channel-pruning-assisted Deep Residual Network for Steganalysis of Digital Images".
Dataset is intended for studying how student programming styles and usage of IDE differs between students who plagiarise their homework and students who solve them honestly.
This data includes 350 Korotkoff sounds and their corresponding osciilometric waveforms, cuff pressure and detected systolic and diastolic pressures.
This MATLAB dataset (.mat) contains the collected real measurement data from a total of 470 access points (APs) deployed in the Linnanmaa campus of the University of Oulu, Finland. The measurements include IDs, dates of data collection, number of users, received traffic data, transmitted traffic data and location names of each AP. Each observation of traffic data and number of users provide the data value at every 10-minute interval between December 18, 2018 and February 12, 2019. Please cite this as: S. P. Sone & Janne Lehtomäki & Zaheer Khan.
E-nose can be used for food authentication and adulteration assessment. Recently, halal authentication has gained attention because of cases of pork adulteration in beef. In this study, The electronic nose was built using nine MQ series gas sensors from Zhengzhou Winsen Electronics Technology Co., Ltd for detection pork adulteration in beef. The list of gas sensors are MQ2, MQ4, MQ6, MQ9, MQ135, MQ136, MQ137, and MQ138. These gas sensors were assembled with an Arduino microcontroller.