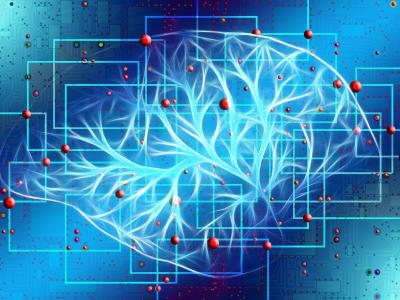
Clean energy technologies, encompassing renewable resources like solar, wind, and hydropower, are essential in the global effort to reduce greenhouse gas emissions and combat climate change. As the globe prepares to transition away from fossil fuels, understanding the factors and parameters influencing the penetration of clean energy into existing energy markets has become a critical step. Controversies surrounding the environmental impacts of renewable technologies, variability in market structures, and economic pressures on clean energy companies can complicate this transition.
- Categories: