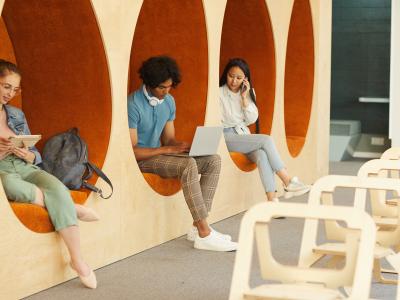
- Categories:
Please cite the following paper when using this dataset:
Vanessa Su and Nirmalya Thakur, “COVID-19 on YouTube: A Data-Driven Analysis of Sentiment, Toxicity, and Content Recommendations”, Proceedings of the IEEE 15th Annual Computing and Communication Workshop and Conference 2025, Las Vegas, USA, Jan 06-08, 2025 (Paper accepted for publication, Preprint: https://arxiv.org/abs/2412.17180).
Abstract:
To download this dataset without purchasing an IEEE Dataport subscription, please visit: https://zenodo.org/records/13896353
Please cite the following paper when using this dataset:
To download the dataset without purchasing an IEEE Dataport subscription, please visit: https://zenodo.org/records/13738598
Please cite the following paper when using this dataset:
N. Thakur, “Mpox narrative on Instagram: A labeled multilingual dataset of Instagram posts on mpox for sentiment, hate speech, and anxiety analysis,” arXiv [cs.LG], 2024, URL: https://arxiv.org/abs/2409.05292
Abstract
This dataset contains audio recordings and transcriptions of toxic speech derived from Indonesian conversations during YouTube videos where scammers are confronted. The dataset captures two separate interactions that escalate into toxic exchanges. Each interaction has been verified by native Indonesian speakers and labeled into two classes: toxic and non-toxic. The dataset includes both the original and preprocessed versions of the speech and text data. The original speech files total 136MB, while the preprocessed speech files are 111,7MB.
UPMVM used three datasets named UD1, UD2 and UD3. UD1 is primarily used to collect and retrieve 280 poetry meters (rhythmic patterns [بحر]) and their corresponding feet. Other uses of this dataset include the design of DFA state function sequences with terminal state information to align the identified verse meters. UD2 is collected from [GitHub - sayedzeeshan/Aruuz] and updated. This update process involves the parsing and tokenization of the UD2 dataset.
Data were collected through the Twitter API, focusing on specific vocabulary related to wildfires, hashtags commonly used during the Tubbs Fire, and terms and hashtags related to mental health, well-being, and physical symptoms associated with smoke and wildfire exposure. We focused exclusively on the period from October 8 to October 31, aligning precisely with the duration of the Tubbs Fire. The final dataset available for analysis consists of 90,759 tweets.
The datasets are used to test an embedding imputation model. There are two different experiments: finance and mobile applications.
Within the Natural Language Processing (NLP) framework, Named Entity Recognition (NER) is regarded as the basis for extracting key information to understand texts in any language. As Bangla is a highly inflectional, morphologically rich, and resource-scarce language, building a balanced NER corpus with large and diverse entities is a demanding task. However, previously developed Bangla NER systems are limited to recognizing only three familiar entities: person, location, and organization.
Food computing is currently a fast-growing field of research. Web mining and content analysis are also increasingly essential in this field, especially for recognising food entities.