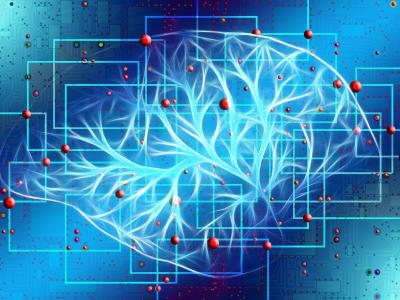
The accelerated development of Machine Learning (ML) tools, combined with broader access to frameworks and infrastructures, has driven the rapid adoption of ML-based solutions in industry. However, their integration into software systems introduces unique challenges, particularly for managing technical debt (TD). While existing frameworks/standards such as Cross-Industry Standard Process for Data Mining (CRISP-DM) and ISO/IEC 5338 provide guidance for ML development, they fail to address the complex interplay of technical and nontechnical factors contributing to TD.
- Categories: