SegPC-2021: Segmentation of Multiple Myeloma Plasma Cells in Microscopic Images
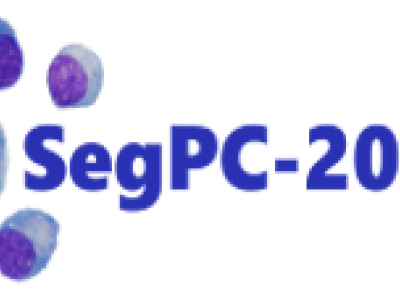
- Citation Author(s):
- Submitted by:
- Anubha Gupta
- Last updated:
- DOI:
- 10.21227/7np1-2q42
- Data Format:
- Links:
- Categories:
- Keywords:
Abstract
Of late, efforts are underway to build computer-assisted diagnostic tools for cancer diagnosis via image processing. Such computer-assisted tools require capturing of images, stain color normalization of images, segmentation of cells of interest, and classification to count malignant versus healthy cells. This dataset is positioned towards robust segmentation of cells which is the first stage to build such a tool for plasma cell cancer, namely, Multiple Myeloma (MM), which is a type of blood cancer. The images are provided after stain color normalization. The problem of plasma cell segmentation in MM is challenging owing to multiple reasons- 1) There is a varying amount of nucleus and cytoplasm from one cell to another. 2) The cells may appear in clusters or as isolated single cells. 3) The cells appearing in clusters may have three cases- (a) cytoplasm of two cells touch each other (b) the cytoplasm of one cell and nucleus of another touch each other, (c) nucleus of cells touch each other. Since the cytoplasm and nucleus have different colors, the segmentation of cells may pose challenges. 4) There may be multiple cells touching each other in the cluster. 5) There may be unstained cells, say a red blood cell underneath the cell of interest, changing its color and shade. 6) The cytoplasm of a cell may be close to the background of the whole image, making it difficult to identify the boundary of the cell and segment it. Hence, the problem is very challenging and interesting. This is an effort towards building an automated pipeline for cancer detection in Multiple Myeloma. The current dataset has 775 images including the above images but captured from two cameras so that researchers can build methods that are invariant to cameras used. Data annotation, both nucleus and cytoplasm are marked separately unlike the previous dataset that had the complete cells as marked. Interested researchers can propose deep learning based or advanced machine learning based solutions for plasma cell segmentation using this dataset.
This data is collected from the subjects suffering from Multiple Myeloma (MM), who came with the symptoms of cancer for diagnosis and/or who are under treatment at the AIIMS, New Delhi, India. Microscopic images were captured from bone marrow aspirate slides of patients diagnosed with MM. MM is a type of white blood cancer, where the plasma cells of blood are involved. Slides were stained using Jenner-Giemsa stain and plasma cells are required to be segmented. Images were captured in raw BMP format using two cameras:
1) with a size of 2040x1536 pixels using cellSens software Version 2.1 (Olympus) attached to the microscope and
2) at a size of 1920x2560pixels from a Nikon camera attached to the microscope.
A total of 775 images are stain color normalized using our in-house methodology. These are divided into the 1) training set of 298 images, 2) Validation set of 200 images, and the test set of 277 images. The dataset was used in the IEEE ISBI 2021 medical image challenge dataset. The leaderboard of the challenge is active. The ground truth of the training and validation dataset are provided, while the GT of the test set will not be shared. The researchers can check the performance on the test dataset by uploading results at the leaderboard at https://segpc-2021.grand-challenge.org/evaluation/final-test-phase/leaderboard/. Full details are available in the readme file.
Instructions:
IMPORTANT:
If you use this dataset, please cite below publications-
- Anubha Gupta, Rahul Duggal, Shiv Gehlot, Ritu Gupta, Anvit Mangal, Lalit Kumar, Nisarg Thakkar, and Devprakash Satpathy, "GCTI-SN: Geometry-Inspired Chemical and Tissue Invariant Stain Normalization of Microscopic Medical Images," Medical Image Analysis, vol. 65, Oct 2020. DOI: https://doi.org/10.1016/j.media.2020.101788. (2020 IF: 11.148)
- Shiv Gehlot, Anubha Gupta and Ritu Gupta, "EDNFC-Net: Convolutional Neural Network with Nested Feature Concatenation for Nuclei-Instance Segmentation," ICASSP 2020 - 2020 IEEE International Conference on Acoustics, Speech and Signal Processing (ICASSP), Barcelona, Spain, 2020, pp. 1389-1393.
- Anubha Gupta, Pramit Mallick, Ojaswa Sharma, Ritu Gupta, and Rahul Duggal, "PCSeg: Color model driven probabilistic multiphase level set based tool for plasma cell segmentation in multiple myeloma," PLoS ONE 13(12): e0207908, Dec 2018. DOI: 10.1371/journal.pone.0207908