ITDAV-25 (Indian Thermal Dataset for Autonomous Vehicles)
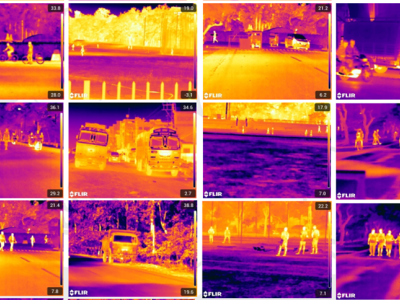
- Citation Author(s):
-
Ayush Kumar Maurya (Department of Chemical Engineeirng, IIT Roorkee)Aryan Sharma (Department of Chemical Engineeirng, IIT Roorkee)Parv Khanna (Department of Chemical Engineeirng, IIT Roorkee)
- Submitted by:
- Parasuraman Sumathi
- Last updated:
- DOI:
- 10.21227/hbje-ef33
- Data Format:
- Categories:
- Keywords:
Abstract
ITDAV-25 (Indian Thermal Dataset for Autonomous Vehicles), a thermal image dataset specifically curated to advance research in Advanced Driver Assistance Systems (ADAS), particularly for environments characterized by low visibility, night-time conditions, and inclement weather. The dataset comprises of 13,688 raw thermal images, collected without any synthetic augmentation techniques. Out of these, 11,501 images are annotated in 2D (38292 number of instances), while the remaining 2,187 images are left unannotated to support tasks such as unsupervised learning or domain adaptation. The annotated portion is divided into 10,132 training images and 1,369 validation images.
Instructions:
The performance of conventional RGB-based datasets tends to degrade significantly under adverse weather conditions such as fog, rain, or low-light scenarios. To address this limitation, we present ITDAV-25 (Indian Thermal Dataset for Autonomous Vehicles), a thermal image dataset specifically curated to advance research in Advanced Driver Assistance Systems (ADAS), particularly for environments characterized by low visibility, night-time conditions, and inclement weather.
ITDAV-25 was captured using the FLIR E54 thermal camera, providing high-resolution thermal imagery (640 × 480 pixels) that accurately represents typical Indian road conditions, including dense traffic and terrain. The dataset has been utilized to train the YOLOv11 object detection model, achieving an impressive mean Average Precision (mAP) of 97.2%.
In total, the dataset comprises 13,688 raw thermal images, collected without any synthetic augmentation techniques. Out of these, 11,501 images are annotated in 2D, while the remaining 2,187 images are left unannotated to support tasks such as unsupervised learning or domain adaptation. The annotated portion is divided into 10,132 training images and 1,369 validation images. Users are free to modify the train/validation split according to their experimental requirements.
All annotations were meticulously performed using the LabelImg tool and are stored in the YOLO format, ensuring compatibility with popular object detection frameworks.
The dataset includes 9 classes as follows:
ID | Class Name |
0 | Person |
1 | Rider |
2 | Bicycle |
3 | Motorcycle |
4 | Car |
5 | Bus |
6 | Stray Animal |
7 | Heavy Vehicle |
8 | Electric Rickshaw |
Kindly refer to README.txt file for further details.