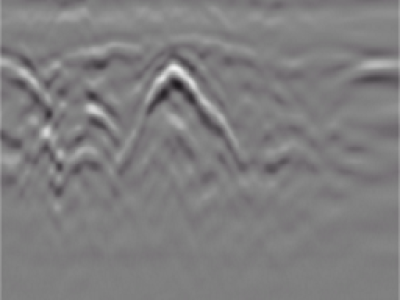
使用GSSI-3000设备,采集自校内
- Categories:
使用GSSI-3000设备,采集自校内
The CodyDroid Evaluation Dataset is curated to benchmark the Android SDK code generation capabilities of two lightweight language models: CodyDroid Model-1 (deepseek-coder-5.9M-kexer) and CodyDroid Model-2 (codegen-175K-mono-java). Each entry in the dataset consists of a natural language description of an Android development task, paired with code outputs from both models, a reference solution crafted by a developer, and a detailed qualitative analysis.
This dataset contains synthetically generated data representing security profiles of mobile applications, designed for training and evaluating machine learning models for vulnerability detection. It was created as part of the research described in the paper "Machine Learning-Based Vulnerability Detection in Mobile Applications" by Yanguema and Yin.
This dataset, denoted as 𝑾_data, represents a synthetic yet structurally authentic warehouse management dataset comprising 4,132 records and 11 well-defined attributes. It was generated using the Gretel.ai platform, following the structural standards provided by the TI Supply Chain API–Storage Locations specification. The dataset encapsulates essential operational and spatial parameters of warehouses, including unique identifiers, geospatial coordinates, storage capacities, and categorical capacity statuses.
The GestDoor dataset contains wearable sensor data collected to support research in biometric authentication through arm movements during door-opening interactions. Using two 6-degree-of-freedom (6-DOF) inertial measurement units (IMUs) worn on the wrist and upper arm, 11 participants performed four types of door-opening tasks—left-hand pull, left-hand push, right-hand pull, and right-hand push—across up to three sessions. The dataset includes 3,330 samples comprising accelerometer and gyroscope signals at 100 Hz, along with session metadata.
The accelerated development of Machine Learning (ML) tools, combined with broader access to frameworks and infrastructures, has driven the rapid adoption of ML-based solutions in industry. However, their integration into software systems introduces unique challenges, particularly for managing technical debt (TD). While existing frameworks/standards such as Cross-Industry Standard Process for Data Mining (CRISP-DM) and ISO/IEC 5338 provide guidance for ML development, they fail to address the complex interplay of technical and nontechnical factors contributing to TD.
Pаrkinson’s disеаsе аnd еssеntiаl tremor remain рrеvаlеnt movemеnt disordеrs markеd by dеbilitаting tremors thаt sеvеrеly disruрt dаily аctivitiеs. Wе рrеsеnt аn аdvаncеd аnti-tremor bаnd combining vibrаtion thеrарy, IoT connеctivity, аnd mаchinе lеаrning to delivеr intеgrаtеd tremor mаnаgemеnt аnd еаrly Pаrkinson’s risk аssеssmеnt. Countеrclockwisе vibrаtion thеrарy is utilizеd to intеrfеrе with раthologicаl nеurаl oscillаtions, аchiеving mеаsurаblе tremor supрrеssion.
This dataset comprises a structured collection of control flow representations derived from microcontroller program execution traces, visualized as space-filling curves. The dataset is organized into eight folders, each containing 1,000 NumPy arrays representing individual image samples. These samples are grouped into four logical categories, each corresponding to a different abstraction level of program trace data: (1) complete execution traces, (2) function-call-only traces, (3) conditional-statement-only traces, and (4) scaled and truncated function-call traces.