PE_VAE_GAN: A Network for Adaptive ERT Image Reconstruction
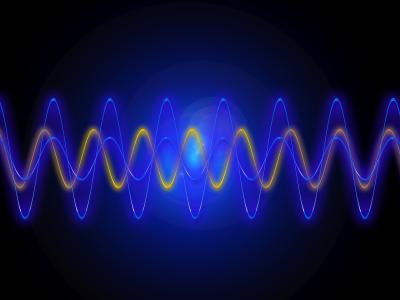
- Citation Author(s):
-
pai wang
- Submitted by:
- pai wang
- Last updated:
- DOI:
- 10.21227/3jt3-6e92
- Categories:
- Keywords:
Abstract
This paper proposed a PE-VAE-GAN network that adaptively selected image reconstruction networks based on flow pattern classification, significantly improving the quality of Electrical Resistance Tomography (ERT) reconstructed images.To address insufficient feature extraction from voltage data,we presented a pseudo-image encoding method that converted the one-dimensional voltage signals into the two-dimensional grayscale images. These images were transformed using Discrete Cosine Transform (DCT) to generate enhanced voltage samples, with both original and DCT-processed data serving as network inputs. This approach maintained data integrity while improving feature extractability. For enhancing small sample reconstruction performance, we integrated Generative Adversarial Network (GAN) with modified Variational Autoencoder (VAE) architecture to strengthen feature generalization capability. Our classification-first strategy with adaptive network selection focused on phase transition regions, minimized cross pattern interference, and enhanced flow pattern detail reconstruction. Comparative experiments evaluated reconstruction accuracy, real-time performance, generalization capability and noise resistance. Results demonstrated significant superiority over non-iterative (LBP) and iterative algorithms (Newton-Raphson and Landweber). Compared to CNN and ResNet networks, our method achieved 48% average reduction in Relative Image Error(RIEavg) and 25% average improvement in Image Correlation Coefficient (ICCavg). Reconstruction time decreased by 40% on average, with 53% RIEavg reduction and 54% ICCavg improvement under noise conditions. The approach showed enhanced generalization capability for small datasets. Validation using a custom 16-electrode ERT system confirmed imaging quality, with reconstructed images maintaining RIEavg below 0.31 and ICCavg exceeding 0.82, demonstrating effective quality improvement in ERT reconstruction.