Event-Related Potentials (P300, EEG) - BCI dataset
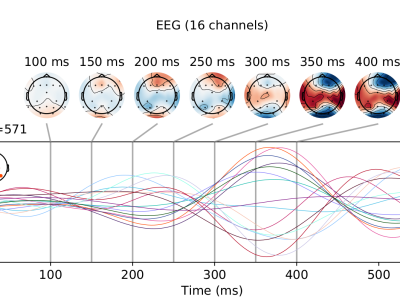
- Citation Author(s):
-
Amin Zollanvari (Nazarbayev University)
- Submitted by:
- Berdakh Abibullaev
- Last updated:
- DOI:
- 10.21227/8aae-d579
- Data Format:
- Links:
- Categories:
- Keywords:
Abstract
The EEG data were acquired from 16 healthy young adults (age range 22 - 30 years) with no neurological, physical, or psychiatric illness history. All the participants were naive BCI users who had not participated in any related experiments before. Informed consent was received from all participants.
Scalp EEG was recorded using 16-channel, active Ag/AgCl electrodes (g.USBamp, g.LADYbird, Guger Technologies OG, Schiedlberg, Austria) with a sampling frequency = 256 Hz. EEG electrodes were positioned according to the International 10-20 system. The right earlobe of participants was used for a ground electrode, whereas the FCz location was used for a reference electrode.
The total number of target letters that a participant had to attend was equal to five. These targets were presented in five consequent sequences with an inter-sequence duration (ISD) of two seconds. In total, five repetitions of each target character were made where one repetition (or trial) consisted of a complete set of 12 random flashes of every row and column (six rows and six columns). The inter-stimulus interval (ISI) was set to 150 ms; likewise, the stimulus duration was 100 ms (i.e., the time length a row/col is highlighted). The minimum time between the same target letter highlights was set to 600 ms, also denoted as a target-to-target (TTI) interval. The stimulus onset asynchrony (SOA) was set to 250 ms. The SOA is the period between starting one stimulus event and the beginning of the next event.
Instructions:
#!/usr/bin/env python3
# -*- coding: utf-8 -*-
"""
Created on Sat May 18 12:57:09 2019
@author: berdakhabibullaev
===================================================
Event Related Potentials (P300 waveform based BCI)
===================================================
Data is converted to MNE python data structure, in a python list.
First, install MNE library via "pip install mne"
You can find more details about the dataset in the following paper:
"Learning Discriminative Spatiospectral Features of ERPs for Accurate Brain-Computer Interfaces."
Abibullaev B, Zollanvari A. IEEE J Biomed Health Inform. 2019 Jan 16. doi: 10.1109/JBHI.2018.2883458.
https://www.ncbi.nlm.nih.gov/pubmed/30668507
"""
# To load the data:
# ==============================
import pickle
filename = 'EEG_data_fileName'
with open(filename, 'rb') as handle:
data = pickle.load(handle)
# Here data is a python list to access first subjects
subject1 = data[0]
print(subject1)
""" Output:
<EpochsArray | 3982 events (all good), 0 - 0.585938 sec, baseline off, ~37.0 MB, data loaded,
'neg': 3413
'pos': 569>
"""
# Extract target and nontarget samples as:
target = subject1['pos']
nonTarget = subject1['neg']
# to get the numpy array:
numpy_array = subject1['pos'].get_data()
#Output: numpy_array.shape()
# trials [x] channels [x] samples
#==============================
# Binary Classification setting:
#=============================
import numpy as np
# construct X,Y dataset for binary classification:
X, Y = [], []
X = np.concatenate([target.get_data(), nonTarget.get_data()])
Y = np.concatenate([np.ones(target.shape[0]), np.zeros(nonTarget.shape[0])])
# Y = 1 => Target Stimulus (a character/letter in the matrix that a user wants to spell)
# Y = 0 => Non-Target Stimulus
"""
==============================
Further info:
==============================
trial duration = 600 milliseconds
sampling frequency = 128 Hz
16 channels
The following pre-processing steps have been done:
1) Detrending
2) Remove bad channels
3) Common-Average Referencing
4) Remove bad epochs
5) Bandpass filtering in the range of [0. 15];
"""
The dataset is useful.
In reply to The dataset is useful. by Yang Liu