GDTM: An Indoor Geospatial Tracking Dataset with Distributed Multimodal Sensors
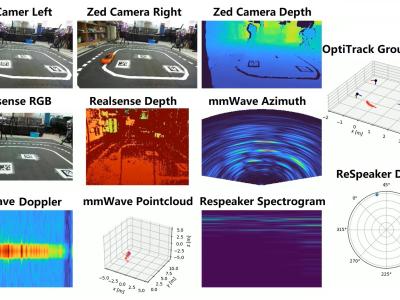
- Citation Author(s):
-
Ho Lyun JeongZiqi WangColin SamplawskiJason WuShiwei FangLance M. KaplanDeepak GanesanBenjamin MarlinMani Srivastava (University of California, Los Angeles)
- Submitted by:
- Ziqi Wang
- Last updated:
- DOI:
- 10.21227/pq3k-2782
- Data Format:
- Research Article Link:
- Categories:
- Keywords:
Abstract
Multimodal sensor fusion has been widely adopted in constructing scene understanding, perception, and planning for intelligent robotic systems. One of the critical tasks in this field is geospatial tracking, i.e., constantly detecting and locating objects moving across a scene. Successful development of multimodal sensor fusion tracking algorithms relies on large multimodal datasets where common modalities exist and are time-aligned, and such datasets are not readily available. Existing multimodal tracking datasets focus mainly on cameras and LiDARs in outdoor environments, while the rich set of indoor sensing modalities is largely ignored. Nevertheless, investigating this tracking problem indoors is non-trivial as it can benefit many applications such as intelligent building infrastructures. Some other datasets either employ a single centralized sensor node or a set of sensors whose positions and orientations are fixed. Models developed on such datasets have difficulties generalizing to different sensor placements. To fill these gaps, we propose GDTM, a nine-hour dataset for multi-modal object tracking with distributed multimodal sensors and reconfigurable sensor node placements. We demonstrate that our dataset enables the exploration of several research problems, including creating multimodal sensor fusion architectures robust to adverse sensing conditions and creating distributed object tracking systems robust to sensor placement variances. A GitHub repository containing the code, data, and checkpoints of this work is available at https://github.com/nesl/GDTM.
Instructions:
Please find detailed instructions and processing scripts in: https://github.com/nesl/GDTM
good