AnxiECG-PPG Database: Synchronized ECG and mobile PPG Signals during Baseline, Physical Activated, and Psychological Activated Conditions
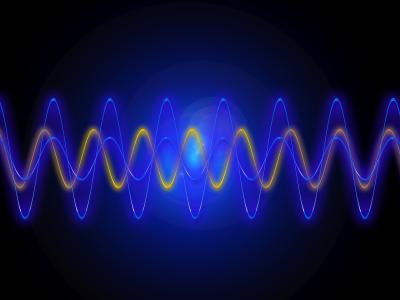
- Citation Author(s):
-
Ana Carolina Almeida (IEETA, Physics Department, University of Aveiro)Francisca Canais (Nevaro Tech)Rita Maçorano (Nevaro Tech)Manuel Lopes (Nevaro Tech)Hugo Alexandre Ferreira (IBEB, Faculty of Sciences of the University of Lisbon)Susana Brás (IEETA, DETI, LASI, University of Aveiro)
- Submitted by:
- Ana Almeida
- Last updated:
- DOI:
- 10.21227/ynse-te33
- Data Format:
- Categories:
- Keywords:
Abstract
The AnxiECG-PPG Database contains synchronized electrocardiogram (ECG) and mobile-acquired photoplethysmography (PPG) recordings from 47 healthy participants. Moreover, the acquisition protocol assesses three distinct states: a 5-minute Baseline, a 1-minute Physical Activated State, and a Psychological Activated state provoked through emotion-induced videos (negative, positive, and neutral emotion valence). Recordings were conducted at the Institute of Electronics and Informatics Engineering of Aveiro and the Institute of Biophysics and Biomedical Engineering from February to March 2024. The BioSignalPlux Explorer Research Kit captured ECG data, while PPG signals were acquired via smartphone (iPhone 11, Apple U.S.) with the HOLI app by NEVARO Tech. This dataset aims to evaluate PPG's potential in deriving cardiac metrics and its viability as an anxiety biomarker, with a comparative analysis against ECG signal recordings.
Instructions:
Background
As defined by the American Psychological Association, anxiety is described as an “emotion characterized by feelings of tension, worried thoughts, and physical changes” [1]. Existing literature indicates that an imbalanced behavioral response, specifically anxiety, leads to hypoactivity in the prefrontal cortex, resulting in a lack of inhibitory neural processes. This imbalance is associated with reduced parasympathetic vagal control, leading to lower heart rate variability (HRV) and higher heart rate (HR) [2]. Currently, anxiety assessment relies heavily on empirical factors, nevertheless, integrating physiological metrics can enhance the precision and effectiveness of treatments. Moreover, the sensing mechanism may be integrated into accessible and portable equipment, such as the smartphone, using the photoplethysmography signal (PPG) opposing the quintessential electrocardiogram (ECG) [3], [4]. While most research in this domain predominantly focuses on ECG-derived cardiac metrics for anxiety evaluation, there is emerging work in exploring the potential of PPG for the same purpose. However, the utilization of a mobile system to acquire PPG signals for anxiety biomarking remains an unexplored avenue.
Methods
Forty-seven healthy participants were recruited for this study. Concerning the data acquired in Aveiro, there was a total of 36 subjects, 21 females and 15 males, with a mean±standard deviation of 22.0±7.8 years old (range: 19–57 years). Regarding the data acquired in Lisbon, it was recruited a total of 11 subjects, 5 females and 6 males, with a mean ± Standard deviation of 25.0±2.3 years old (range: 22–29 years). This study was approved by the Ethics and Deontology Council of the University of Aveiro (32-CED/2023 and 59-CED/2023), and informed consent was attained from all the participants.
To acquire the ECG signal, it was used the 4-channel BioSignalPlux Explorer Research Kit from Plux [5]. Two of the three ECG electrodes were placed in each wrist and the reference electrode was placed above the pelvic bone. The sensor’s reading digitalization was made through the software OpenSignals which receives via Bluetooth the acquired physiological signals with a sampling frequency of 1000Hz, this signal was further processed, and the R-peaks were identified with the Neurokit2 toolbox using a 5th-order high-pass Butterworth filter with a cut-off frequency of 0.5Hz and powerline filtering (50 Hz). To acquire the PPG signal, a Smartphone (iPhone 11, Apple U.S.) with the HOLI app by NEVARO Tech was used [6]. The system outputted the PPG signal and identified its peaks, sampled at 100Hz.
As a methodology to induce different emotional valence, arousal, and anxiety states, emotion-inducing videos priorly validated by the team were used [7], [8]. A set of five emotions was selected: anger, fear, and disgust (all of negative valence and shown to be effective in inducing anxiety); amusement (positive valence); and neutral state (used as control). The neutral video consisted of a jittering white cross (duration: 60s), while the negative valence videos included scenes of: domestic violence (duration: 65s); Bear Grylls eating a larva (duration: 62s); and a horror video “Lights Out” (duration: 147s). The positive valence video included scenes from a Doritos commercial (duration: 59s). The neutral video was repeated in between excerpts.
During the study protocol, participants remained seated during a 5-minute Baseline acquisition. After, they were asked to stand up and provoke Physical Activation using verbal communication and physical movements involving jumping and skipping for 1 minute. Ensuing, the participants seated and continued with a 5-minute data collection (only the first minute was used to describe the peak physical activation). Following, participants were asked to focus on the videos. This represented approximately 14 minutes of Psychological Activation.
Data description
This database contains simultaneous ECG and PPG recordings from 47 participants acquired in two different locations, the sociographic data is in the file “sociographic_data.txt” containing ID code, sex (Female – F, Male - M), age, and data acquisition place.
Within the dataset of each signal in the .pkl files (“ECG.pkl” and “PPG.pkl”) there are the signal’s recordings identified by the participant’s code and divided by the protocol’s epochs (“State”): “Baseline”, “Physical Activation”, “Happy”, “Neutral_1”, “Fear”, “Neutral_2”, “Anger”, “Neutral_3”, “Disgust”. These file formats are compatible with the Python pickle package, making it easy to import the data [9].
Usage notes
These data are intended to be used to explore similarities between ECG and PPG-derived cardiac metrics. Furthermore, the data describes physiological responses to psychological activations of negative valence allowing it to additionally be intended to be used to explore relationships between cardiac metrics (from both signals) and the Autonomic Nervous System, namely during anxiety states. The data also comprises physiological responses during control states of positive and neutral valence, and physically activated state. A present limitation of the data is a high level of noise associated with both the ECG signal (due to wrist placement) and PPG signal (due to undesired movements consequent of tiredness and abrupt reactions by participants), which allows for the present dataset to be used in signal qualifier algorithm development and studies associated with the role of signal’s noise.
All data provided in this work is intended solely for scientific investigation purposes and is strictly prohibited from any form of commercial use. Reproduction, distribution, or any other use of the data for commercial purposes is expressly forbidden without prior written consent from the copyright holder.
Acknowledgements
This database was acquired as part of a master's thesis in Biomedical Engineering at the University of Aveiro, Portugal in collaboration with NEVARO Tech. The author thanks the Institute of Electronics and Informatics Engineering of Aveiro and the Institute of Biophysics and Biomedical Engineering for providing the facilities for data acquisition.
CONFLICTS OF INTEREST
There are no conflicts of interest regarding this project.
Bibliography
[1] American Psychological Association, “Anxiety,” https://www.apa.org/topics/anxiety.
[2] J. F. Thayer and R. D. Lane, “A model of neurovisceral integration in emotion regulation and dysregulation,” J Affect Disord, vol. 61, no. 3, pp. 201–216, Dec. 2000, doi: 10.1016/S0165-0327(00)00338-4.
[3] S. Blok, M. A. Piek, I. I. Tulevski, G. A. Somsen, and M. M. Winter, “The accuracy of heartbeat detection using photoplethysmography technology in cardiac patients,” J Electrocardiol, vol. 67, pp. 148–157, Jul. 2021, doi: 10.1016/J.JELECTROCARD.2021.06.009.
[4] Z. Wang et al., “Heart rate variability in generalized anxiety disorder, major depressive disorder and panic disorder: A network meta-analysis and systematic review,” J Affect Disord, vol. 330, pp. 259–266, Jun. 2023, doi: 10.1016/J.JAD.2023.03.018.
[5] D. Batista, H. P. da Silva, A. Fred, C. Moreira, M. Reis, and H. A. Ferreira, “Benchmarking of the BITalino biomedical toolkit against an established gold standard,” Healthc Technol Lett, vol. 6, no. 2, p. 32, 2019, doi: 10.1049/HTL.2018.5037.
[6] NEVARO, “Holi - NEVARO,” NEVARO. Accessed: Mar. 26, 2024. [Online]. Available: https://www.nevaro.tech/holi/
[7] R. das N. A. Maçorano, “Exploratory Psychometric Validation and Efficacy Assessment Study of Social Phobia Treatment based on Augmented and Virtual Reality Serious Games and Biofeedback Dissertação orientada por,” Master Thesis, Universidade de Lisboa, Lisboa, 2020. Accessed: Mar. 26, 2024. [Online]. Available: http://hdl.handle.net/10451/47968
[8] M. F. S. da L. Canais, “Exploratory Psychometric Validation and Efficacy Assessment Study of an Agoraphobia Treatment based on Virtual Reality Serious Games and Biofeedback,” Master Thesis, Universidade de Lisboa, Lisboa, 2020. Accessed: Mar. 26, 2024. [Online]. Available: http://hdl.handle.net/10451/48441
[9] G. Van Rossum, “The Python Library Reference, release 3.8.2.” Python Software Foundation, 2020.
Please give me access to your database, I will use it in my research related to mental disorder detection using multimodal signals.