Experiments Dataset for PerfCam: Digital Twinning for Production Lines Using 3D Gaussian Splatting and Vision Models
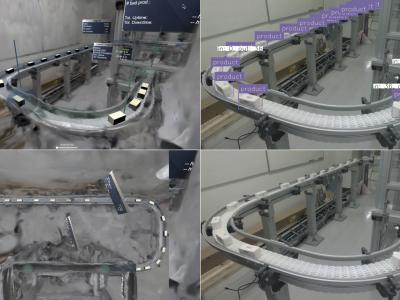
- Citation Author(s):
-
Michel Gokan Khan
(School of Engineering Sciences, KTH Royal Institute of Technology, Stockholm, Sweden)
Renan Guarese(School of Electrical Engineering and Computer Science, KTH Royal Institute of Technology, Stockholm, Sweden)
Fabian Johnson (AstraZeneca)Xi Wang(School of Industrial Engineering and Management, KTH Royal Institute of Technology, Stockholm, Sweden)
- Submitted by:
- Michel Gokan
- Last updated:
- DOI:
- 10.21227/73cd-3668
- Data Format:
- Categories:
- Keywords:
Abstract
Dataset for PerfCam: Digital Twinning for Production Lines Using 3D Gaussian Splatting and Vision Models
KTH Royal Institute of Technology, SCI; AstraZeneca, Sweden Operations
Michel Gokan Khan, Renan Guarese, Fabian Johonsson, Xi Vincent Wang, Anders Bergman, Benjamin Edvinsson, Mario Romero Vega, Jérémy Vachier, Jan Kronqvist
PerfCam is an open-source proof of concept that integrates 3D Gaussian Splatting with real-time object detection to achieve precise digital twinning of industrial production lines. This approach leverages existing camera systems for both 3D reconstruction and object tracking, reducing the need for additional sensors and minimizing initial setup and calibration efforts.
This repository presents the dataset used in the PerfCam's original paper. This dataset is to support further research in the area of industrial 3D reconstruction, digital twinning, and predictive maintenance.
Paper's Abstract:
We introduce PerfCam, an open source Proof-of-Concept (PoC) digital twinning framework that combines camera and sensory data with 3D Gaussian Splatting and computer vision models for digital twinning, object tracking, and Key Performance Indicators (KPIs) extraction in industrial production lines. By utilizing 3D reconstruction and Convolutional Neural Networks (CNNs), PerfCam offers a semi-automated approach to object tracking and spatial mapping, enabling highly accurate digital twins that capture real-time KPIs such as availability, performance, Overall Equipment Effectiveness (OEE), and rate of conveyor belts in the production line. We validate the effectiveness of PerfCam through a practical deployment within realistic test production lines in the pharmaceutical industry and contribute an openly published dataset to support further research and development in the field. The results demonstrate PerfCam’s ability to deliver actionable insights through its precise digital twin capabilities, underscoring its value as an effective tool for developing usable digital twins in smart manufacturing environments and extracting operational analytics.
Instructions:
3D Reconstruction Dataset
- Dataset Generated From PerfCam's Robotic Camera:
-
experiments/az_kul_small_line/3d_reconstruction/by_perfcam/
-
- Images Taken Using A Pixel 7 Pro:
-
experiments/az_kul_small_line/3d_reconstruction/by_phone
-
Experiment at AZ Kul
- Details of the Experiment and Events:
-
experiments/az_kul_small_line/object_and_event_detection
-
- Dataset for Trained YOLO Model:
-
experiments/az_kul_small_line/object_and_event_detection/trained
-
COLMAP Point Clouds
- COLMAP Workspace:
-
experiments/az_kul_small_line/3d_reconstruction/by_perfcam/trained/colmap
-
3D Gaussian Splats
- 3D Gaussian Splats Based on PerfCam:
-
experiments/az_kul_small_line/3d_reconstruction/by_perfcam/trained/SuGaR/dn_consistency/output
-
- 3D Gaussian Splats Based on Pixel 7 Pro:
-
experiments/az_kul_small_line/3d_reconstruction/by_phone/trained/SuGaR
-
Statistics, Figures, CSV Results, and Related Scripts to Parse
- Scripts to Parse the Dataset:
-
experiments/az_kul_small_line/object_and_event_detection/scripts
-
- Figures:
-
experiments/az_kul_small_line/object_and_event_detection/stats/figures
-
- CSVs for Object Detection and KPI Extraction:
-
experiments/az_kul_small_line/object_and_event_detection/stats/csv
-
License
All files in this repository are licensed under the Apache-2.0 License except the YOLO weights under experiments/*/object_and_event_detection/trained/model/train/weights
, which are licensed under the AGPL (look for a LICENSE-YOLO-AGPL inside the folder next to the weight files).
Citing PerfCam Dataset
If you use PerfCam or PerfCam Dataset in your research, please use the following BibTeX entry.
@article{perfcam,
title={PerfCam: Digital Twinning for Production Lines Using 3D Gaussian Splatting and Vision Models},
author={Michel Gokan Khan and Renan Guarese and Fabian Johonsson and Xi Vincent Wang and Anders Bergman and Benjamin Edvinsson and Mario Romero Vega and Jeremy Vachier and Jan Kronqvist},
journal={TBA},
year={2025}
}
@data{73cd-3668-25,
doi = {10.21227/73cd-3668},
url = {https://dx.doi.org/10.21227/73cd-3668},
author = {Gokan Khan, Michel and Guarese, Renan and Johnson, Fabian and Wang, Xi and Romero, Mario and Vachier, Jérémy and Kronqvist, Jan},
publisher = {IEEE Dataport},
title = {Experiments Dataset for PerfCam: Digital Twinning for Production Lines Using 3D Gaussian Splatting and Vision Models},
year = {2025}
}