Timezone-Aware Auto-Regressive Long Short-Term Memory Model for Multi-Pollutant Prediction
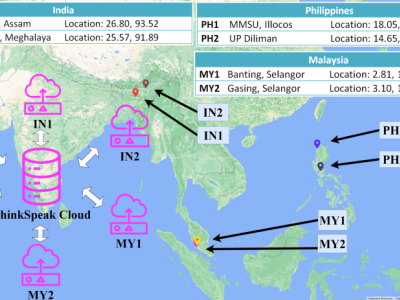
- Citation Author(s):
- Submitted by:
- SHUBHANKAR MAJUMDAR
- Last updated:
- DOI:
- 10.21227/d0j1-1c78
- Data Format:
- Research Article Link:
- Links:
- Categories:
- Keywords:
Abstract
The data used in this work is collected using the AirBox Sense system developed to detect six air pollutants, ambient temperature, and ambient relative humidity. The pollutants are Nitrogen Dioxide (NO2), surface Ozone (O3), Carbon Monoxide (CO), Sulphur Dioxide (SO2), Particulate Matter (PM2.5, and PM10). The sensors monitor these pollutants in real-time and store them in a cloud-based platform using a cellular module. Data are collected every 20 seconds, producing 4320 readings each day. Data instances collected from July 2022 to December 2022 are used as training and validation data. To validate, the trained model is used to predict the pollution for seven days (01 January 2023 to 07 January 2023). Six sensors were deployed in geologically separate locations
Instructions:
Download the zip file and use the .csv format dataset.
Data Characteristics
- Temporal Resolution: The data is recorded at 15-minute intervals, offering detailed temporal resolution.
- Missing Data: Both datasets contain missing values due to sensor malfunctions or communication issues. These missing values were handled using imputation techniques as part of the preprocessing phase.
- Pollutants Measured: The datasets include the following pollutants:
- NO2 (Nitrogen Dioxide)
- O3 (Ozone)
- CO (Carbon Monoxide)
- SO2 (Sulfur Dioxide)
- PM2.5 (Particulate Matter ≤ 2.5 micrometers)
- PM10 (Particulate Matter ≤ 10 micrometers)