Time Series Nowcasting of Wind Speed using Tree based Ensemble Methods
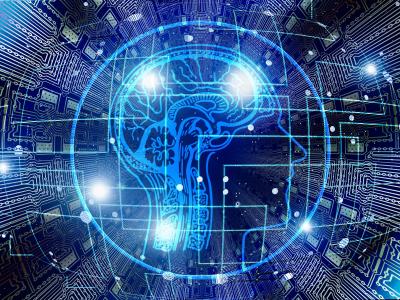
- Citation Author(s):
- Submitted by:
- PARUL ARORA
- Last updated:
- DOI:
- 10.21227/wjak-kv58
- Data Format:
- Categories:
- Keywords:
Abstract
With the advent of huge data availability and cheap processing power to derive insights from data ,applications of Big Data and Machine Learning are gaining popularity in every industry. Now, Predicting about future is no more an alternate but a necessity to increase efficiency with accurate output. Forecasting for a shorter duration (Nowcasting) fits perfectly in this space to estimate the final production for better planning and control. The ever increasing renewable energy production in the world has led to the stipulation of forecasting techniques for their integration in electrical grids.There are many forecasting methods but in this paper, decision tree based ensemble models are discussed. Ensembles combine the several weaker models and extract the different information which results in skillful and robust models. For the application of ensemble methods, univariate time series wind forecasting is performed using Global Energy Forecasting Competition-2014 data. Application of Various newly developed ensemble methods like CatBoost, LightGBM, and XGBoost is shown for predicting wind speed .These methods along with existing Random Forest(RF) and Conventional Gradient Boosting Machines(CGBM) are applied on this dataset, and it has been shown that the proposed new methods perform better than the RF and CGBM in terms of accuracy and speed.
Instructions:
This file contains the univariate wind speed data which is used in Global Energy Forecasting Competition (GEFCom-14).