TDDPG: Temporal-Constrained DDPG-Based Autonomous Navigation for eVTOLs in Dynamic Environments
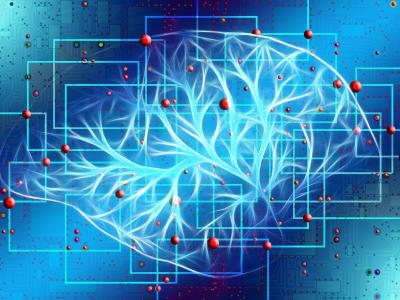
- Citation Author(s):
-
Mingyu Qi
- Submitted by:
- mingyu qi
- Last updated:
- DOI:
- 10.21227/5gbw-m962
- Data Format:
- Categories:
- Keywords:
Abstract
With the introduction of the low-altitude economy concept, the application of electric vertical takeoff and landing (eVTOL) aircraft has become more widespread, particularly in search and rescue missions. However, most of the existing path planning methods cannot effectively cope with dynamic environments and changes in destinations, which limits the ability of eVTOL drones to autonomously perform planning tasks in unknown dynamic environments. To address these challenges, this paper first proposes a Temporal-constrained Deep Deterministic Policy Gradient (TDDPG) reinforcement learning framework that integrates timing constraints to solve efficient exploration and path planning problems in dynamic environments through continuous action space. Then, a multi-objective composite reward function is designed, which comprehensively considers various obstacles and environmental factors, improving the success rate of eVTOL path planning. Finally, to tackle the convergence challenges of TDDPG, this paper introduces initialization exploration and prioritized experience replay mechanisms, effectively enhancing learning efficiency in dynamic environments. Simulation results show that TDDPG can successfully complete the eVTOL path planning task in an unknown dynamic obstacle environment. Compared with other algorithms, TDDPG obtains higher rewards in fixed endpoint tasks and shows better efficiency and success rate in random endpoint tasks.
Instructions:
Training result