RAW-Q: Raw Water Quality Dataset, Thailand
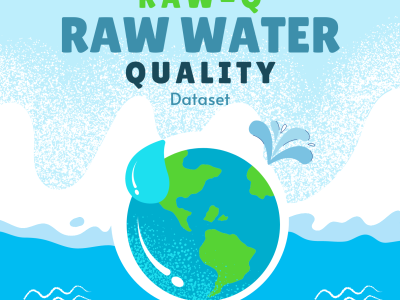
- Citation Author(s):
-
Jaturapith Krohkaew
(Department of Big Data Management and Analytics, Rajamangala University of Technology Thanyaburi)
Pongpon Nilaphruek(Department of Big Data Management and Analytics, Rajamangala University of Technology Thanyaburi)
Niti Witthayawiroj(Department of Computer Science, Rajamangala University of Technology Thanyaburi)
Sakchai Uapipatanakul (Kinetics Corporation Ltd, Thailand) - Submitted by:
- Jaturapith Krohkaew
- Last updated:
- DOI:
- 10.21227/3q8d-jw96
- Categories:
- Keywords:
Abstract
The significance of having sustainable water quality data cannot be overstated. It plays a crucial role in comprehending the historical variations and patterns in river conditions and also helps in understanding how industrial waste impacts the well-being of aquatic ecosystems. To achieve sustainable water management practices, it is imperative to rely on dependable and extensive data. Therefore, accurate monitoring and assessment of various water quality parameters become essential.
In this context, we introduce a reconstructed daily water quality dataset that complements scarce historical observations at six station points along the Chao Phraya River in Thailand. Through the use of Internet of Things (IoT) technology, we reconstructed this dataset for the period from June 2022 to February 2023. The recorded parameters encompass Turbidity, Optical Dissolved Oxygen, Dissolved Oxygen Saturation, Spatial Conductivity, Acidity/Basicity, Total Dissolved Solids, Salinity, Temperature, Chlorophyll, and Depth, each collected from six different stations.
This comprehensive dataset comprises a total of 211,322 data points, which have been organized into six CSV files. To evaluate the dataset, we employed the Long Short-Term Memory (LSTM) algorithm. The results of this evaluation are expected to offer valuable insights to researchers studying river ecosystems, empowering them to make informed decisions and foster sustainable water management practices.
Instructions:
This dataset consists of six csv files, each of which has sheet structures as described below:
s1 Logs.csv,
s2 Logs.csv,
s3 Logs.csv,
s4 Logs.csv,
s5 Logs.csv,
s15 Logs.csv.