CV-MuSeNet Complex-Valued Multi-Signal Segmentation: Dataset
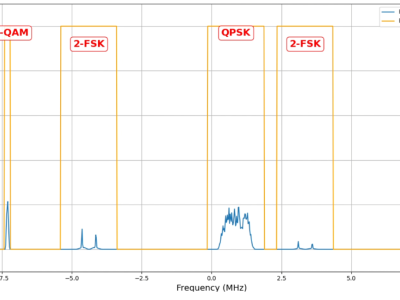
- Citation Author(s):
-
Sangwon Shin
- Submitted by:
- Sangwon Shin
- Last updated:
- DOI:
- 10.21227/wv2n-tv20
- Data Format:
- Categories:
- Keywords:
Abstract
The increasing congestion of the radio frequency spectrum presents challenges for efficient spectrum utilization. Cognitive radio systems enable dynamic spectrum access with the aid of recent innovations in neural networks. However, traditional real-valued neural networks (RVNNs) face difficulties in low signal-to-noise ratio (SNR) environments, as they were not specifically developed to capture essential wireless signal
properties such as phase and amplitude. This work presents CMuSeNet, a complex-valued multi-signal segmentation network for wideband spectrum sensing, to address these limitations. Extensive hyperparameter analysis shows that a naive conversion of existing RVNNs into their complex-valued counterparts is ineffective. Built on complex-valued neural networks (CVNNs) with a residual architecture, CMuSeNet introduces a complexvalued Fourier spectrum focal loss (CFL) and a complex plane intersection over union (CIoU) similarity metric to enhance training performance. Extensive evaluations on synthetic, indoor overthe-air, and real-world datasets show that CMuSeNet achieves an average accuracy of 98.98%-99.90%, improving by up to 9.2 percentage points over its real-valued counterpart and consistently outperforms state of the art. Strikingly, CMuSeNet achieves the accuracy level of its RVNN counterpart in just two epochs, compared to the 27 epochs required for RVNN, while reducing training time by up to a 92.2% over the state of the art. The results highlight the effectiveness of complex-valued architectures in improving weak signal detection and training efficiency for spectrum sensing in challenging low-SNR environments.
Instructions:
This dataset includes synthetic AWGN IQ samples and Indoor-Over-the-air (OTA) dataset for CMuSeNet