CMU-SynTraffic-2022
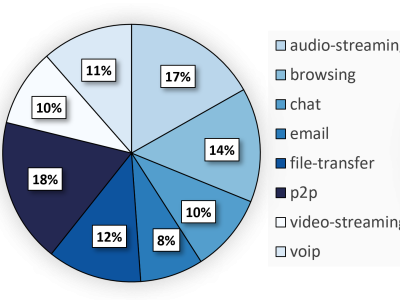
- Citation Author(s):
- Submitted by:
- Drake Cullen
- Last updated:
- DOI:
- 10.21227/wc3q-jz97
- Data Format:
- Categories:
- Keywords:
Abstract
Anonymous network traffic is more pervasive than ever due to the accessibility of services such as virtual private networks (VPN) and The Onion Router (Tor). To address the need to identify and classify this traffic, machine and deep learning solutions have become the standard. However, high-performing classifiers often scale poorly when applied to real-world traffic classification due to the heavily skewed nature of network traffic data. Prior research has found synthetic data generation to be effective at alleviating concerns surrounding class imbalance, though a limited number of these techniques have been applied to the domain of anonymous traffic detection. A CTGAN, CopulaGAN, VAE, and SMOTE were utilized to create viable synthetic anonymous network traffic samples. Ultimately, we amalgamate the data generated by the GANs, VAE, SMOTE, and real traffic from the CIC-Darknet2020 dataset into a comprehensive dataset, CMU-SynTraffic-2022, for future research on synthetic data and anonymous network traffic.
Instructions:
The synthetic portion of this dataset consists of 432,847 SMOTE, 700,000 CTGAN, 700,000 CopulaGAN and 700,000 VAE samples. CMU-SynTraffic-2022 also contains 117,620 real samples from CIC-Darknet2020 [Lashkari] for a total of 2,650,467 samples. In addition to the 64 features present in CIC-Darknet2020, this dataset also contains the data source label (real, CTGAN, CopulaGAN, VAE, SMOTE). Since this dataset is comprised mostly of synthetic data, training machine learning classifiers on the entire dataset could result in overfitting to the data. Care should be taken to the proportions of synthetic and real data used for training and testing. CMU-SynTraffic-2022 is intended for future examination and application of synthetic network traffic data.
A. Habibi Lashkari, G. Kaur, and A. Rahali, “DIDarknet: A Contemporary Approach to Detect and Characterize the Darknet Traffic using Deep Image Learning,” 2020 the 10th International Conference on Communication and Network Security. ACM, Nov. 27, 2020 [Online]. Available: http://dx.doi.org/10.1145/3442520.3442521