EMGNet: An EMG Dataset for Neural Decoding
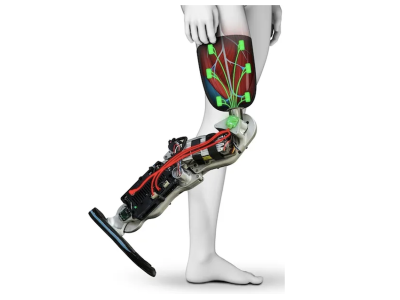
- Citation Author(s):
- Submitted by:
- Brokoslaw Laschowski
- Last updated:
- DOI:
- 10.21227/3n4y-7y55
- Links:
- Categories:
- Keywords:
Abstract
Surface electromyography (EMG) can be used to interact with and control robots via intent recognition. However, most machine learning algorithms used to decode EMG signals have been trained on small datasets with limited subjects, impacting their generalization across different users and tasks. Here we developed EMGNet, a large-scale dataset for EMG neural decoding of human movements. EMGNet combines 7 open-source datasets with processed EMG signals for 132 healthy subjects (152 GB total size). We include manually annotated data for four muscles (i.e., tibialis anterior, medial gastrocnemius, rectus femoris, and biceps femoris) and six locomotor tasks, including standing, level-ground walking, stair ascent and descent, and ramp ascent and descent. Each dataset included in EMGNet was modified to achieve a consistent data structure for ease of use and to establish a standardized pipeline for signal processing and machine learning. The mission of EMGNet is to provide a large-scale, open-source platform to support the development and comparison of next-generation EMG neural decoding algorithms for research in neuroscience and robotics.
Instructions:
*Details are provided in the ReadMe file. Email Dr. Laschowski (brokoslaw.laschowski@utoronto.ca) for questions and/or technical assistance.
ok
Looks great! It's a shame you have to be an IEEE member or have a subscription to access this.
ok