Weakly Supervised Semantic Segmentation in Histopathology Based on Global Proportions
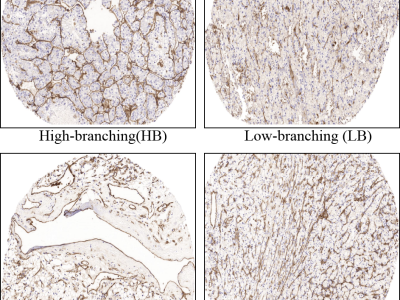
- Citation Author(s):
- Submitted by:
- Yangping Li
- Last updated:
- DOI:
- 10.21227/egfr-0211
- Data Format:
- Categories:
- Keywords:
Abstract
This dataset comprises 325 histopathological images of clear cell renal cell carcinoma (ccRCC) tissue sections, designed to characterize vascular morphology based on CD31 immunohistochemical staining. Each image was scanned at 10× magnification and annotated with global proportions for three distinct vascular patterns: high-branching (HB), low-branching (LB), and sinusoidal (SN). The provided annotations include the relative distribution of each vascular class per image.
The primary purpose of this dataset is to support the development of weakly supervised semantic segmentation methods, where only global category proportions are available rather than pixel-level annotations. Researchers can use this dataset to build models capable of predicting pixel-level vascular pattern segmentation aligned with the provided global labels. It facilitates advances in computational pathology, particularly in scenarios requiring learning from limited supervision.
Instructions:
The dataset accompanies the article Weakly Supervised Semantic Segmentation in Histo-pathology Based on Global Proportions and consists of image data and corresponding annotations.
Dataset Composition
This dataset comprises 325 histopathological images of clear cell renal cell carcinoma (ccRCC) tissue sections. The goal is to capture and quantify the heterogeneity of vascular morphology using immunohistochemically stained slides.
Each image was annotated with global proportions of three vascular patterns: high-branching (HB), low-branching (LB), and sinusoidal (SN).
Unlike datasets that include pixel-wise labels, this collection provides image-level annotations only, making it suitable for weakly supervised learning settings. The global vascular proportions per image are provided in the gt_prob.csv file. This structure enables training and evaluation of models that perform pixel-level semantic segmentation from global supervision signals.
Image Acquisition
Tissue sections were prepared from ccRCC samples as tissue microarrays (TMAs). Sections were cut at a thickness of 4 µm using a microtome under controlled conditions at 4°C to preserve tissue morphology. Immunohistochemical staining targeting CD31 was performed using the Medac autostainer 480S.
Slides were scanned with the Leica Aperio GT 450 DX whole-slide scanner, achieving a pixel resolution of approximately 0.5 µm per pixel. After scanning, images were manually inspected by trained personnel. Cores with out-of-focus regions, staining artifacts, tissue breakage, or other quality deficiencies were excluded to maintain dataset integrity.
Annotation and Preprocessing
From the scanned whole-slide images, individual cores were extracted and processed at 10× magnification. To address variability in staining and ensure consistency across samples, Vahadane’s stain normalization technique was applied to all images.
A board-certified pathologist with over 20 years of experience reviewed each core and annotated the global proportion of each vascular pattern. Class frequencies are distributed as follows: high-branching (145 images), low-branching (97 images), and sinusoidal (83 images). For the training set, each image was assigned a class label based on its dominant vascular pattern. If the two leading pattern proportions were within 20% of each other, the image was labeled as non-predominant to represent mixed morphological cases.
This dataset enables researchers to develop models for weakly supervised semantic segmentation, particularly in domains where dense annotations are unavailable. It supports methods such as proportion-supervised learning, multiple instance learning, and other weakly supervised frameworks, and can be used to evaluate how well pixel-level predictions align with global label distributions in histological images.