Virtual Cycling Load
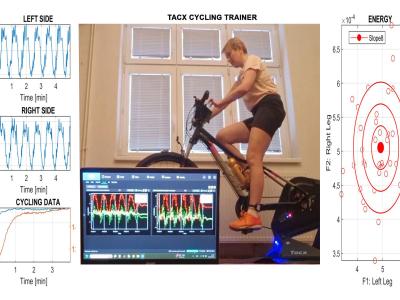
- Citation Author(s):
-
Daniel Martynek (University of Chemistry and Technology, ICO: CZ60461373)Alexandra Molcanova (University of Chemistry and Technology, ICO: CZ60461373)
- Submitted by:
- Ales Prochazka
- Last updated:
- DOI:
- 10.21227/vjfa-1b64
- Data Format:
- Research Article Link:
- Categories:
- Keywords:
Abstract
Motion analysis provide important information in rehabilitation, performance evaluation, and movement symmetry assessment, with applications including neurology, biomedicine, surgery, and sports monitoring. The integration of wearable sensors and signal processing forms a robust interdisciplinary platform for such analysis. Specific methods are based on monitoring physiological and motion responses during controlled exercises that simulate real-world motion scenarios. This study focuses on processing of signals from wearable sensors collected from smart indoor trainers, enabling motion monitoring under predefined load conditions. The acquired datasets include heart rate (HR), motion accelerometric signals, and fitness parameters (cycling speed). The research objectives include analysis of motion patterns, evaluation of motion symmetry under varying loads, and examination of heart rate responses to load variations. Signal processing is conducted using advanced methods that include computational intelligence, digital signal processing, and artificial intelligence tools for data classification. Results point to the mean delay of the HR drop to 97\% of the HR range in 15s after the change from the cycling on the slope of 8% to the rest period and the following drop to 5% in next 54s. The classification of spectral features evaluated separately for the left and right legs pointed the classification accuracy of 93.7% estimated by the use of the two layer neural network and the symmetry coefficient of 1.1 for the slope of 8%. In general, the paper presents selected processing methods and experimental results pointing to the effectiveness of computational intelligence in motion analysis.
Instructions:
The first dataset includes XLSX tables of seven experiments of accelerometric data recorded with the sampling frequency of 100 Hz for 5 different slopes (-8, -4, 0, +4, +8%). The second dataset includes heart rate data acquired during 5 experiments, each consisting of a 2-minute period of cycling on the selected slope followed by a 2-minute period of rest.