Unlearnable Data
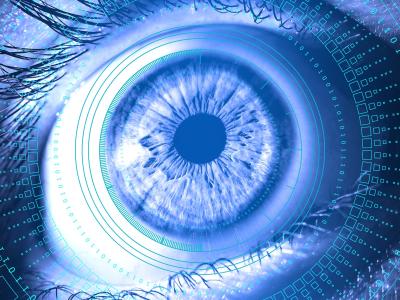
- Citation Author(s):
-
Thushari HapuarachchiKaiqi Xiong
- Submitted by:
- Thushari Hapuarachchi
- Last updated:
- DOI:
- 10.21227/bvcb-8p38
- Categories:
- Keywords:
Abstract
Making images unlearnable through imperceptible perturbations is to prevent unauthorized image scraping from training deep neural networks (DNNs). Most existing methods for breaking these unlearnable data focus on applying image transformation techniques to disrupt the added perturbations, with limited attention given to modifying the classification tasks (target classes) of DNNs as an alternative approach. In this paper, we explore the vulnerabilities of unlearnable data, focusing mainly on modifying the classification tasks rather than applying image transformation techniques. We first experimentally demonstrate that DNNs can learn from unlearnable data by modifying their classification tasks and then propose a divide and conquer framework for breaking unlearnable data. The proposed framework breaks unlearnable data by decomposing a given classification task into simple classification tasks, which is robust to unlearnable data, and performing the given classification task using the simplified tasks. To find the optimal way to decompose the classification task, we propose both a manual approach and an automated approach based on genetic algorithms. We further apply nonlinear transformations to unlearnable data to make the DNNs in our framework more robust to the unlearnable data. After evaluating our framework on breaking six prominent unlearnable CIFAR-10 datasets, we achieve a test accuracy similar to clean data (more than 85%), highlighting the vulnerability of existing unlearnable data. This study shows that existing unlearnable data must be improved in order to prevent DNNs from learning by modifying the classification tasks.
Instructions:
Data are in numpy array (.npy) format. Please load the data accordingly.