SPRSound: Open-Source SJTU Paediatric Respiratory Sound Database
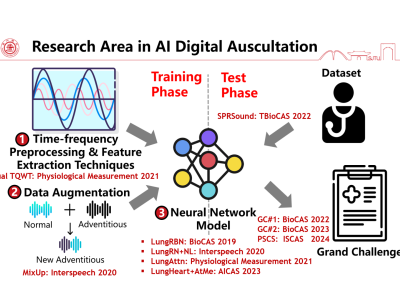
- Citation Author(s):
-
Qing Zhang (Shanghai Jiao Tong University)Jing Zhang (Shanghai Engineering Research Center of Intelligence Pediatrics (SERCIP))Jiajun Yuan (Shanghai Engineering Research Center of Intelligence Pediatrics (SERCIP))Huajie Huang (Shanghai Jiao Tong University)Yuhang Zhang (Shanghai Jiao Tong University)Baoqin Zhang (Taicang Affiliated Hospital of Soochow University)Gaomei Lv (Linyi City People Hospital)Shuzhu Lin (Fengcheng Hospital)Na Wang (Linyi City Maternal and Child Health Hospital)Xin Liu (Fifth Affiliated Hospital of Harbin Medical University)Mingyu Tang (Shanghai Children’s Medical Center)Yahua Wang (Shanghai Children’s Medical Center)Hui Ma (Shanghai Children’s Medical Center)Lu Liu (Shanghai Children’s Medical Center)Shuhua Yuan (Shanghai Children’s Medical Center)Hongyuan Zhou (Shanghai Tuoxiao Intelligent Technology Co., Ltd)Jian Zhao (Shanghai Jiao Tong University)Yongfu Li (Shanghai Jiao Tong University)Yong Yin (Shanghai Engineering Research Center of Intelligence Pediatrics (SERCIP))Liebin Zhao (Shanghai Engineering Research Center of Intelligence Pediatrics (SERCIP))Guoxing Wang (Shanghai Jiao Tong University)Yong Lian (Shanghai Jiao Tong University)
- Submitted by:
- Yongfu Li
- Last updated:
- DOI:
- 10.21227/nfkk-1x47
- Research Article Link:
- Links:
- Categories:
- Keywords:
Abstract
It has proved that the auscultation of respiratory sound has advantage in early respiratory diagnosis. Various methods have been raised to perform automatic respiratory sound analysis to reduce subjective diagnosis and physicians’ workload. However, these methods highly rely on the quality of respiratory sound database. In this work, we have developed the first open-access paediatric respiratory sound database, SPRSound. The database consists of 2,683 records and 9,089 respiratory sound events from 292 participants. Accurate label is important to achieve a good prediction for adventitious respiratory sound classification problem. A custom-made sound label annotation software (SoundAnn) has been developed to perform sound editing, sound annotation, and quality assurance evaluation. A team of 11 experienced paediatric physicians is involved in the entire process to establish golden standard reference for the dataset.
Instructions:
Our database is the first open access respiratory sound database in the pediatric population, aging from 1 month to 18 years old. The respiratory sounds contained in the dataset were recorded at the pediatric respiratory department at Shanghai Children’s Medical Center (SCMC) using Yunting model II Stethoscopes. The recordings are saved in .wav format with naming rules as follows: Each name is composed of 5 elements separated with underscores, including the patient number, age, gender, the recording location, and the recording number of the participants. 1. Patient number (e.g., 65101170) 2. Age (e.g., 0.4) 3. Gender a. Male (0) b. Female (1) 4. Recording location a. left posterior (p1) b. left lateral (p2) c. right posterior (p3) d. right lateral (p4) 5. Recording number (e.g., 3246) The annotations at the record and event level are provided in this database. At the record level, each recording with poor signal quality was annotated as Poor Quality, while the recordings with high signal quality were annotated as Normal, CAS, DAS, or CAS & DAS according to the presence/absence of continuous/discontinuous adventitious respiratory sounds. At the event level, each recording was segmented into multiple respiratory event and annotated as Normal, Rhonchi, Wheeze, Stridor, Coarse Crackle, Fine Crackle, or Wheeze+Crackle. The annotation information of each recording is saved in .json format with the same filename, which contains the annotation at record level and event level. The annotation at record level is Normal, CAS, DAS, CAS & DAS or Poor Quality. The annotation at event level consists of the start (ms) and the end (ms) of respiratory events, and the corresponding type of respiratory events (Normal, Rhonchi, Wheeze, Stridor, Coarse Crackle, Fine Crackle, Wheeze+Crackle). An example of annotation file is as follow: ```json { "recording_annotation": "Normal", "event_annotation": [ { "start": 342, "end": 2515, "type": "Normal" }, { "start": 2557, "end": 3776, "type": "Normal" }, { "start": 4547, "end": 5651, "type": "Normal" }, { "start": 6439, "end": 8065, "type": "Normal" }, { "start": 8363, "end": 9201, "type": "Normal" } ] } ```
Good evening, I'm speaking to you from Argentina. I would like to use your database to test the model I built for detecting wheezing. I would greatly appreciate it if you could help me with the database.