RGB-NIR Flame dataset
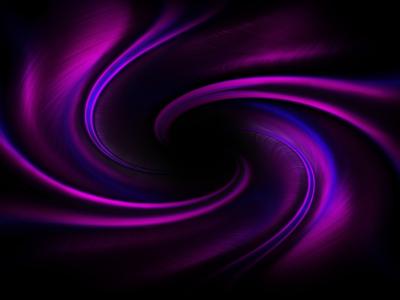
- Citation Author(s):
-
Dai Jinyang
- Submitted by:
- Qixing Zhang
- Last updated:
- DOI:
- 10.21227/8ppn-gy81
- Links:
- Categories:
- Keywords:
Abstract
We propose a high-quality dual-channel (RGB-NIR) flame dataset to address the issues of low resolution, insufficient diversity, limited annotation quality, and scarcity of multispectral data in existing flame detection datasets. The dataset is constructed using a dual-camera acquisition platform, capturing high-resolution synchronized RGB-NIR image pairs that encompass seasonal variations in flame morphology. It also includes challenging non-flame samples such as vehicle headlights, streetlamps, and sunlight reflections to enhance discrimination capability in real-world scenarios. All images undergo rigorous modality registration and are annotated in PASCAL VOC format.
Instructions:
Competition Tasks
Participants are encouraged to develop models for:
- Flame Detection: Accurately identify flames in both RGB and NIR spectra.
- False Alarm Suppression: Distinguish flames from challenging non-flame objects.
Dataset Usage Guidelines
- Training Phase: Use only the provided training set (14,806 pairs).
- Testing Phase: Submit predictions on the test set (3,171 pairs) for evaluation.
- Preprocessing: Participants may apply alignment, normalization, or augmentation, but must disclose methods.
- External Data: Only pretrained models on public datasets (e.g., ImageNet) are allowed; no additional flame data permitted.