OSM-MEPS data for solar PV modeling
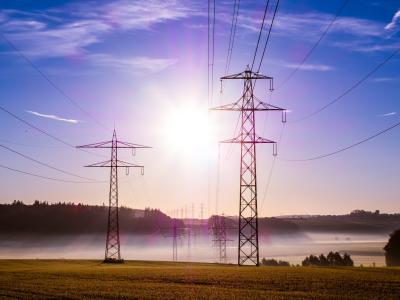
- Citation Author(s):
- Submitted by:
- PETER MUTUKU
- Last updated:
- DOI:
- 10.21227/k6p8-mf68
- Links:
- Categories:
- Keywords:
Abstract
Open source modeling has emerged as a transformative paradigm in energy and power
systems studies by enabling transparent, reproducible and collaborative development of tools and models.
The scarcity of high-resolution input data, coupled with unlocalized modeling, poses a significant challenge
to progress toward sustainable energy. This paper presents a systematic method for modeling energy and
power systems by integrating key procedural steps with first-principles thinking, the scientific method and
the engineering design process. The scientific method—encompassing observation, hypothesis formulation,
experimentation and analysis—ensures empirical rigor, while the engineering design process—centered
on problem definition, solution development and iterative evaluation—prioritizes technical feasibility and
scalability. The proposed systematic Open Source Modeling Method for Energy and Power Systems (OSMMEPS)
was implemented to develop a solar photovoltaic (PV) energy model. High-resolution irradiance and
weather data for the year 2024—such as direct normal irradiance and diffuse horizontal irradiance, which
are crucial for assessing solar energy potential—were sourced from the Solcast-DNV company at a spatialtemporal
resolution of 90 meters and 5 minutes. The solar PV energy model yielded 1,626 MWh/year
and was compared with an established PV energy system model (pvlib-python) which produced 1,120
MWh/year, the Global Solar Atlas (GSA) estimate of 1,513 MWh/year and the real, site-measured energy
generation of 1,631 MWh/year from the Serres-C solar PV plant in Greece. The OSM-MEPS RMSE is
584.62 kWh and R² is 0.887, compared to pvlib’s RMSE of 1526.11 kWh and R² of 0.232. This consistent
advantage indicates OSM-MEPS’s suitability for annual-scale forecasting, particularly in systems with
diverse seasonal behavior. In Adelaide, pvlib failed to track real energy measurements altogether, with an
R² of –0.959. At the same time, the OSM-MEPS model captured variance with an R² of 0.866, indicating
a strong fit for the irradiance and input physics variables. A solar rooftop PV energy feasibility case study
was conducted for the Njagu-Mhasibu-Ruiru Estate in Kenya. The OSM-MEPS model yielded 14,329.45
kWh/year, compared to pvlib’s 10,048 kWh/year and the Global Solar Atlas (GSA) estimate of 12,784
kWh/year at 8.75 kW solar PV installed capacity.
Instructions:
Use Jupyter Notebook after installing Python
Dataset Files
- GLEN_0_DEC_2024.csv (Size: 2.21 KB)
- kisumu.csv (Size: 4.45 KB)
- GLEN_sorted_2.csv (Size: 20.04 KB)
- PVOutput_Data.csv (Size: 49 bytes)
- serres_2024_full_dataset.csv (Size: 7.09 KB)
- PVOutput_Data.xlsx (Size: 4.83 KB)
- vpnt.csv (Size: 5.64 KB)
- GLEN_sorted.csv (Size: 21.94 KB)
- kisumu_sorted.csv (Size: 4.34 KB)
- weather_data.csv (Size: 71.28 KB)
- vnpt3_sorted.csv (Size: 1.44 KB)
- csv_-1.11665_36.92927_fixed_23_0_PT5M_2024.csv (Size: 8.24 MB)
- csv_-34.9599769_138.6414601_fixed_22.5_270_PT5M.csv (Size: 9.98 MB)
- csv_40.886273_23.912687_fixed_23_180_PT5M.csv (Size: 8.62 MB)
- csv_-1.11665_36.92927_fixed_23_0_PT5M.csv (Size: 8.24 MB)
- csv_-25.78950982_28.3069343_fixed_30_0_PT5M.csv (Size: 9.57 MB)
- csv_-0.085477_34.718851_fixed_23_0_PT5M.csv (Size: 10.07 MB)