OneToMany_ToolSynSeg
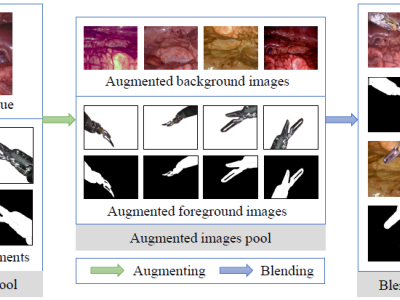
- Citation Author(s):
-
An Wang (CUHK)Mobarakol Islam (UCL)Mengya Xu (NUS)Hongliang Ren (CUHK)
- Submitted by:
- An Wang
- Last updated:
- DOI:
- 10.21227/7fda-2d79
- Data Format:
- Research Article Link:
- Links:
- Categories:
- Keywords:
Abstract
Data diversity and volume are crucial to the success of training deep learning models, while in the medical imaging field, the difficulty and cost of data collection and annotation are especially huge. Specifically in robotic surgery, data scarcity and imbalance have heavily affected the model accuracy and limited the design and deployment of deep learning-based surgical applications such as surgical instrument segmentation. Considering this, we rethink the surgical instrument segmentation task and propose a one-to-many data generation solution that gets rid of the complicated and expensive process of data collection and annotation from robotic surgery. In our method, we only utilize a single surgical background tissue image and a few open-source instrument images as the seed images and apply multiple augmentations and blending techniques to synthesize amounts of image variations. In addition, we also introduce the chained augmentation mixing during training to further enhance the data diversities. The proposed approach is evaluated on the real datasets of the EndoVis-2018 and EndoVis-2017 surgical scene segmentation. Our empirical analysis suggests that without the high cost of data collection and annotation, we can achieve decent surgical instrument segmentation performance. Moreover, we also observe that our method can deal with novel instrument prediction in the deployment domain. We hope our inspiring results will encourage researchers to emphasize data-centric methods to overcome demanding deep learning limitations besides data shortage, such as class imbalance, domain adaptation, and incremental learning.
Instructions:
Details about how to generate and use the data are available at https://github.com/lofrienger/Single_SurgicalScene_For_Segmentation.
Please visit the github repository for reference.