CMTMU Dataset — Comprehensive multi-type and multi-unit appliance dataset
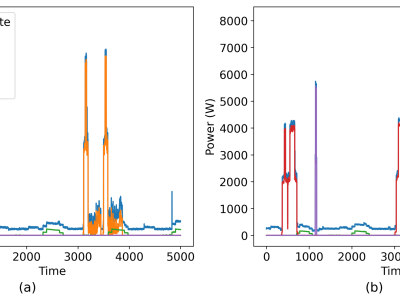
- Citation Author(s):
-
Louyang Yu (Nanjing University of Information Science and Technology)Gaozhenyang Wang (Nanjing University of Information Science and Technology)Zhengju Ren (Nanjing University of Information Science and Technology)Konglin Zhu (the Department of Electrical and Computer Engineering, Michigan State University)Yadang Chen (Nanjing University of Information Science and Technology)Alex Liu ( Midea Group)
- Submitted by:
- Wenbin Yu
- Last updated:
- DOI:
- 10.21227/4key-ng14
- Data Format:
- Categories:
- Keywords:
Abstract
To advance real-world applications of non-intrusive load monitoring (NILM), we propose the CMTMU dataset—an innovative dataset that simulates realistic residential power consumption scenarios involving both multiple appliance types and multiple units of the same type. Existing NILM datasets largely overlook such complexity, limiting model generalizability. The CMTMU dataset is constructed by applying an offset-overlay technique to REFIT data, enabling the simulation of concurrent multi-unit appliance usage. Furthermore, a margin-based event detection mechanism and threshold-based labeling strategy are used to annotate appliance states with high fidelity. CMTMU includes diverse appliance categories such as fridges, kettles, washing machines, and dishwashers, capturing realistic load variations and operational overlaps. Experimental results using state-of-the-art NILM models demonstrate that the CMTMU dataset significantly enhances the training and evaluation of models under complex appliance operation conditions, thereby promoting more accurate load disaggregation and appliance quantity identification in smart energy systems.
Instructions:
The CMTMU dataset is a crucial resource for non - intrusive load monitoring (NILM) research, specifically designed to address the challenges of identifying multiple appliances in a household setting. It contains power consumption data that simulates scenarios where residents with similar usage habits operate multiple identical appliances simultaneously. This dataset is constructed based on the REFIT load dataset, leveraging an offset - superposition method to generate multi - appliance operation scenarios.The dataset is primarily intended for researchers working on NILM algorithms. It can be used to train and evaluate models for identifying the operating states of individual appliances from the total household power consumption signal. For example, machine learning and deep learning models can be trained on this dataset to predict whether an appliance is on or off at a given time.The dataset is also useful for developing methods to identify the number of identical appliances operating simultaneously. This is a challenging task in NILM, and the CMTMU dataset provides the necessary data to test and improve algorithms for this purpose.