IEEE Brain Data Bank Hackathon at COMPSAC 2018 Conference
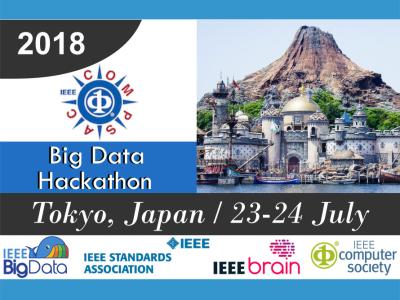
- Submission Dates:
-
to
- Citation Author(s):
-
J.A. AngueraJ. BoccanfusoJ.L. RintoulO. Al-HashimiF. FarajiJ. JanowichE. KongY. LarraburoC. RolleE. JohnstonA. Gazzaley
- Submitted by:
- Sin-Kuen Hawkins
- Last updated:
- DOI:
- 10.21227/H2P38M
- Categories:
- Keywords:
Abstract
Instructions:
We invite teams (3-4 member teams) of data scientists, computer scientists, engineers, statisticians, analysts, and problem solvers to explore new patterns or knowledge from the given NeuroRacer datasets.
You can develop data mashup scheme based on use cases to cross reference different datasets and apply statistical analysis, visualization, and machine learning tools to statistically analyze and develop predictive models for what changed between “shoot only or single tasking” and “drive & shoot or multi-tasking” from the EEG (electroencephalography) signals. Think outside the box and come up with innovative ideas that bring more value out of the data, or choose one or more of the following questions.
Beginner Challenge Questions:
- What are the strengths vs. limitations of the EEG technology? How do consumer-level EEG headsets compare to laboratory-grade equipment?
- What are the realistic EEG applications in daily life (automatic driving, interactive games, Internet Marketing, etc.)? Provide convincing prototypes (virtual or real).
- Try to conduct an event-related potential (ERP) analysis of the data in one or more conditions. How does this approach compare to that used in the Nature paper (i.e., ERSP-Event-Related Spectral Perturbation or time-frequency analysis)? Hint: check out the EEGLab and Fieldtrip tutorial
Advanced Challenge Questions:
-Try conducting an ICA decomposition analysis of the data (Hint: this is best done in EEGLab). How does this approach compare to that used in the Nature paper or the ERP analysis suggested above? What new information can we learn using this approach?
-Would a micro-state analysis be appropriate for the data? What new knowledge might we learn from such an approach?
-What advanced methods (e.g., deep learning, but also others) are available that would help predict post-game performance? Specifically by what mechanisms and by how much?
A subset sample dataset is available under "Documentation". For the full datasets, please submit a request form on the bottom of this page.
For more details, go to https://bigdatawg.nist.gov/bdgmm_compsac2018.html