Arabic Dialectal Diagnostic (ADD)
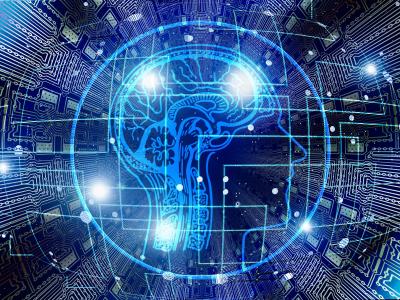
- Citation Author(s):
-
Jinane MounsefAli Raza
- Submitted by:
- Jinane Mounsef
- Last updated:
- DOI:
- 10.21227/tcnv-c593
- Data Format:
- Links:
- Categories:
- Keywords:
Abstract
Accurate diagnosis of patient conditions becomes challenging for medical practitioners in urban metropolitan cities. A variety of languages and spoken dialects impedes the diagnosis achieved through the exploratory journey a medical practitioner and patient go through. Natural language processing has been used in well-known applications, such as Google Translate, as a solution to reduce language barriers. Languages typically encountered in these applications provide the most known, used or standardized dialect. The Arabic language can benefit from the common dialect, which is available in such applications. However, given the diversity of dialects in Arabic, in the healthcare domain, there is a risk associated with incorrect interpretation of a dialect, which can impact the diagnosis or treatment of patients. Arabic language dialect corpuses published in recent research work can be applied to rule natural language-based applications. Our study aims to develop an approach to support medical practitioners by ensuring that the diagnosis is not impeded based on the misinterpretation of patient responses. Our initial approach reported in this work adopts the methods used by practitioners in the diagnosis carried out within the scope of the Emirati and Egyptian Arabic dialects. In this paper, we develop and provide a public dataset, known as the Arabic Dialectal Diagnostic (ADD) dataset, which is a corpus of audio samples related to healthcare. To train machine learning models, the dataset development is designed with multi- class labelling. Our work indicates that there is a clear risk of bias in datasets, which may come about when a large number of classes do not have enough training samples. Our crowd sourcing solution presented in this work may be an approach to overcome the sourcing of audio samples. Models trained with this dataset may be used to support the diagnosis made by medical practitioners.
Instructions:
The recordings are saved according to the question and statement that are presented to the user. As an example here, a user chooses the Emirati dialect. This triggers a random question “Who do you live with?” and the random statement “I live with my wife” to be presented to the user. Once the recording is complete and uploaded in the corpus, it is presented and labeled as: “Emirati_Q_who_do_you_live_with_S_i_live_with_my_wife _L_spouse”. The underscore (’_’) delimiter is used to separate the fields of interest for automation in dataset processing later. The first field is the dialect, followed by Q to indicate the question, S to indicate the statement and finally L to indicate the labels associated with the audio sample.