UTAUT2 Dataset on Gen Z Use of Short Learning Videos
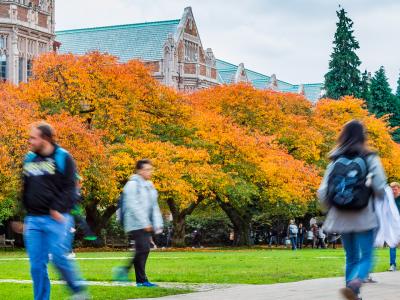
- Citation Author(s):
- Submitted by:
- Khaled Halimi
- Last updated:
- DOI:
- 10.21227/akyj-3y82
- Data Format:
- Categories:
- Keywords:
Abstract
This dataset contains raw survey data collected from 207 Generation Z students at the University of Guelma, Algeria. The data was gathered via an online questionnaire to investigate the adoption of short educational videos for academic purposes in higher education, based on an extended Unified Theory of Acceptance and Use of Technology 2 (UTAUT2) model.
The dataset includes responses for variables representing the following constructs from the extended UTAUT2 model and related factors:
- Performance Expectancy (PE)
- Effort Expectancy (EE)
- Social Influence (SI)
- Habit (HT)
- Hedonic Motivation (HM)
- Price Value (PV)
- Facilitating Conditions (FC)
- Personalization (PERS)
- Behavioral Intention (BI)
- Use Behavior (UB)
Demographic information for participants is also included:
- Age
- Gender
- Education Level
- Faculty / Specialty
Instructions:
Instructions on How to Utilize the Dataset
This dataset (ShortVideoAdoptionData.csv) provides raw survey responses for investigating Generation Z students' adoption of short educational videos. To effectively utilize this dataset, please follow these steps:
- Access and Download: Download the ShortVideoAdoptionData.csv file and the accompanying README.md file from the IEEE DataPort repository.
- Understand the Data Structure and Variables:
- Open the README.md file. This file serves as the data dictionary.
- Carefully review the "Data Structure" section to understand how the data is organized (rows = participants, columns = variables).
- Consult the "Data Dictionary" section for a detailed description of each column header in the CSV file. This includes:
- Demographic and control variables (e.g., Age, Gender, Specialty).
- Survey items (e.g., PE1, EE3, HT4, PERS1, BI2, UB1) that measure the theoretical constructs of Performance Expectancy (PE), Effort Expectancy (EE), Social Influence (SI), Habit (HT), Hedonic Motivation (HM), Price Value (PV), Facilitating Conditions (FC), Personalization (PERS), Behavioral Intention (BI), and Use Behavior (UB).
- Note the measurement scale used for the survey items (5-point Likert scale from 1=Strongly Disagree to 5=Strongly Agree).
- Load the Data into Software:
- Open the ShortVideoAdoptionData.csv file using your preferred statistical software (e.g., SPSS, R, Python pandas, SmartPLS, Excel, etc.).
- Ensure the software correctly interprets the comma separation and column headers.
- Perform Data Preparation (as needed for your analysis):
- Missing Data: Check for any missing values in the dataset. The original study used listwise deletion for analysis variables; you may choose to apply the same or an alternative justified method depending on your analytical approach.
- Composite Score Calculation: For analyses using construct scores (e.g., in regression or as reflective constructs in PLS-SEM where they are treated as composites), calculate the mean of the relevant items for each construct as listed in the Data Dictionary. For example, a composite score for Performance Expectancy (PE) would typically be the average of items PE1, PE2, PE3, PE4, PE5, PE6, PE7, PE8, and PE9.
- Conduct Analyses:
- Refer to the associated publication for detailed information on the study's research questions, theoretical model (Figures 1 and 3 in the paper), and analytical methods.
- Descriptive Statistics: Calculate descriptive statistics (means, standard deviations) for the calculated composite scores or individual items, and frequency counts/percentages for demographic variables, similar to the study's results.
- Measurement Model Assessment: If conducting PLS-SEM or similar SEM, assess the reliability and validity of the measurement model using the relevant items for each construct. Evaluate indicator loadings, construct reliability (e.g., Cronbach's Alpha, Composite Reliability), convergent validity (e.g., Average Variance Extracted - AVE), and discriminant validity (e.g., HTMT). Compare your findings to the study's results.
- Structural Model Assessment: Analyze the hypothesized relationships between constructs (direct paths) based on the model presented in the associated paper (Figures 1 and 3). Report path coefficients and their significance (e.g., using bootstrapping in PLS-SEM). Evaluate the model's explanatory power (R²) and predictive relevance (Q²). Compare your results to the study's findings.
- Moderation Analysis: Investigate potential moderating effects of demographic variables (Age, Gender, Specialty, Education Level) on the relationships between constructs, as performed in the original study's Multi-Group Analysis (MGA). Refer to the paper's results section for specific relationships tested.
- Interpret Findings: Interpret your analysis results in the context of the UTAUT2 model and Gen Z characteristics, comparing them to the interpretations and conclusions drawn in the associated publication.
By following these instructions and referencing the README.md file and the associated publication, you can effectively load, prepare, and analyze this dataset to explore the factors influencing Gen Z's adoption of short educational videos.