Traffic Flow Dataset for China’s Congested Highways & Expressways
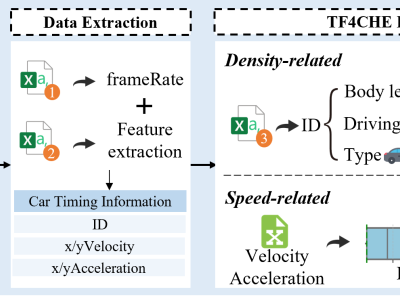
- Citation Author(s):
- Submitted by:
- hongrui kou
- Last updated:
- DOI:
- 10.21227/wfy1-xx38
- Data Format:
- Categories:
- Keywords:
Abstract
The Traffic Flow Dataset for China’s Congested Highways & Expressways (TF4CHE) is derived from AD4CHE (Aerial Dataset for China's Congested Highways & Expressways). AD4CHE collects data using unmanned aerial vehicles (UAVs) operating at an altitude of 100 meters and employs advanced calibration techniques to achieve a positioning accuracy of approximately 5 cm. It provides comprehensive vehicle metrics, including position, speed, classification, as well as unique parameters such as self-offset and yaw rate. AD4CHE covers highway and expressway data across 11 distinct scenarios in five cities in China, comprising a total of 68 data segments. Each data segment includes three files: xx_recordingMeta.csv, xx_tracks.csv, and xx_tracksMeta.csv, which respectively provide video metadata, vehicle trajectories, and trajectory metadata. However, AD4CHE is a pre-development dataset, and its raw parameters are not directly applicable to traffic flow time-series forecasting tasks. TF4CHE is constructed by processing the original frame rate data from AD4CHE into sequential data and applying theoretical formulations from the rail transit domain. It serves as an application-oriented dataset suitable for direct use in research on traffic flow prediction and congestion identification.
Instructions:
Participants will engage with two primary challenges designed to test different aspects of traffic analysis capabilities. The first task focuses on short-term traffic flow prediction, where competitors will develop models to forecast three key parameters: vehicle density k(t), average traffic speed v(t), and traffic flow q(t). These predictions must be generated for three distinct time horizons: 15 seconds, 30 seconds, and 45 seconds into the future, testing both immediate and medium-range forecasting abilities.
The second task centers on traffic congestion identification, requiring participants to create sophisticated systems capable of classifying congestion levels into four categories: low, medium, high, and full congestion. Beyond simple classification, solutions must also predict congestion probability P(t) as a continuous value between 0 and 1, and demonstrate the ability to identify recurring congestion patterns within the dataset. This dual-task structure ensures comprehensive evaluation of both predictive and analytical capabilities.
For the traffic flow prediction task, submissions will be evaluated using three standard metrics: Mean Absolute Error (MAE), Mean Squared Error (MSE), and Root Mean Squared Error (RMSE). These metrics will be calculated separately for each predicted parameter and time horizon, providing a detailed assessment of prediction accuracy across different scenarios.