Robust pavement planar coefficients estimation from LiDAR point clouds
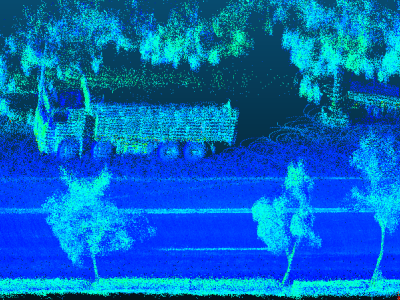
- Citation Author(s):
-
Zheng Zou
- Submitted by:
- Zheng Zou
- Last updated:
- DOI:
- 10.21227/re5p-0188
- Data Format:
- Links:
- Categories:
- Keywords:
Abstract
Pavement planar coefficients are critical for a wide range of civil engineering applications, including 3D city modeling, extraction of pavement design parameters, and assessment of pavement conditions. However, existing plane fitting methods often struggle to maintain accuracy and stability in complex road environments, particularly when the point cloud is affected by non-pavement objects such as trees, curbstones, pedestrians, and vehicles. This paper presents REoPC, a robust two-stage estimation method based on road point clouds acquired using hybrid solid-state LiDAR. The approach consists of two main parts: coarse estimation and refined estimation. Part I develops a dual-plane sliding window to filter out major outliers and extract a coarse plane. Part II constructs a new cost function based on the Geman-McClure function to further mitigate residual noise and reduce fitting instability caused by outlier influence and algorithmic randomness. REoPC is evaluated on both synthetic and real-world datasets under diverse conditions. In addition to outperforming mainstream methods in accuracy and robustness across both typical and challenging scenarios, REoPC also demonstrates low sensitivity to parameter variations, highlighting its practical reliability across a broad range of operating conditions.
Instructions:
Please use "CloudCompare" to open it.
CloudCompare