Meteorological Data for Kampala, Entebbe, Masindi and Namulonge observation Stations
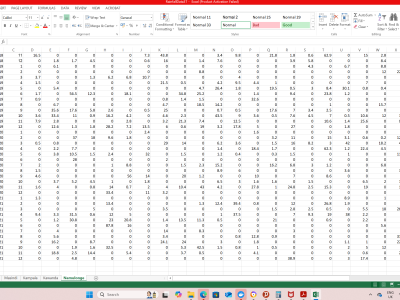
- Citation Author(s):
-
BALIKUDDEMBE JOSEPH KIWUUWA (UGANDA NATIONAL METEOROLOGICAL AUTHORITY)
- Submitted by:
- BALIKUDDEMBE KIWUUWA
- Last updated:
- DOI:
- 10.21227/h2v7-gk59
- Data Format:
- Links:
- Categories:
- Keywords:
Abstract
This study explores the application of the
Long Short-Term Memory (LSTM) neural network architecture
to develop a machine learning model capable of predicting
the onset, cessation, and duration of rainfall seasons. Climate
observation data from weather stations in Entebbe, Kampala,
Kawanda, Namulonge, and Masindi were used. The dataset
includes 12,025 daily records from 1991 to 2021, consisting of
surface pressure, temperature, wind speed, relative humidity, and
rainfall amounts. Two main prediction tasks were addressed:
binary classification of daily rainfall occurrence (rainfall or no
rainfall), and regression-based prediction of rainfall amounts.
The data were split into 80% for training and 20% for testing
across both tasks. Classification model performance metrics
included accuracy, precision, recall, specificity, F1 score, and
Matthews correlation coefficient (MCC), while the regression
model was evaluated using mean absolute error (MAE). The
classification model achieved an accuracy of 0.915, precision
of 0.9435, recall of 0.9166, specificity of 0.864, F1 score of
0.9299, and MCC of 0.7653. The developed seasonality model
was validated using the March–April–May 2025 rainfall season
and demonstrated the capability to detect and predict seasonal
transitions. These findings hold significant potential for the
advancement of early warning systems aimed at forecasting
rainfall patterns, which are critical for managing weather-related
hazards and reducing risks to life and property.
Index Terms—Long Short-Term Memory (LSTM), Rainfall
Onset, Rainfall Cessation, Stacked LSTM, Numerical Weather
Prediction System (NWPS), Matthews Correlation Coefficient
(MCC), Mean Absolute Error (MAE), Relative Humidity (RH)
Instructions:
The excel file contains files for each of the regions contained in each folder.
Kampala, Entebbe, Namulonge and Masindi
The file names are named according to the data contained like Rainfall, temperature, relative humidity.
Rows represent days and columns, columns calendar days like 01st 01 1999.
Referring to 01st of Janaury 1999.