Hart Rate Varibilityb (HRV) Parameters for ECG Signal and Upper Limb Movement Dataset
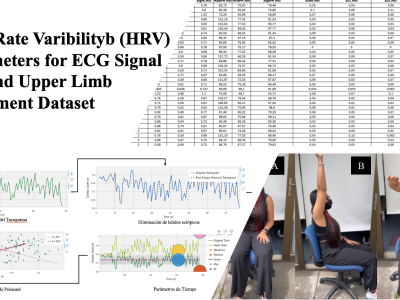
- Citation Author(s):
- Submitted by:
- Camila Clavijo Montes
- Last updated:
- DOI:
- 10.21227/3qfr-vx56
- Data Format:
- Categories:
- Keywords:
Abstract
Heart Rate Variability (HRV) parameters provide valuable insights into the autonomic nervous system’s regulation of the cardiovascular system in response to various physiological conditions, particularly during dynamic tasks. This dataset consists of ECG recordings obtained during the execution of three dynamic tasks based on the three-dimensional movement of the upper limb. The data is available in both .XLSX and .CSV formats, containing 166 rows and 21 columns, all of which correspond to HRV parameters such as RR intervals, heart rate, and frequency-domain measures. These parameters include MinRR, AvgRR, SDNN, LF/HF ratio, and power spectral components, offering a comprehensive view of cardiovascular responses during physical exertion and recovery. The dataset is designed to support the development of AI models aimed at various applications, including rehabilitation, sports performance monitoring, and predictive health systems. By utilizing this database, researchers and practitioners can develop machine learning algorithms to enhance personalized health monitoring, optimize athletic training, and contribute to advancements in human-machine interaction.
Instructions:
The HRV Upper Limb Movement Database is available for download in both .XLSX and .CSV formats on IEEE DataPort. It contains 166 rows and 21 columns, with each row representing a recording during dynamic upper limb movements, and each column corresponding to a specific Heart Rate Variability (HRV) parameter. The parameters include MinRR, AvgRR, MaxBPM, MinBPM, AvgBPM, SDNN, LF/HF Ratio, and power spectral components such as ULF, VLF, LF, and HF. These parameters provide detailed information about heart rate variability in response to physical activity, allowing for analysis of autonomic nervous system regulation, exertion, and recovery. This dataset is particularly useful for developing AI models aimed at health monitoring, rehabilitation, sports performance analysis, and predictive health systems. By leveraging these HRV parameters, researchers and practitioners can create machine learning algorithms that enhance the understanding of cardiovascular responses to upper limb movement, contributing to advancements in personalized health management and performance optimization.
To access the HRV Upper Limb Movement Database, users can visit IEEE DataPort, where the dataset is available for download in both .XLSX and .CSV formats. Ensure you have an IEEE DataPort account or necessary permissions to download the dataset.