BengaliSent140 - A Bengali Hate Speech Fusion Dataset
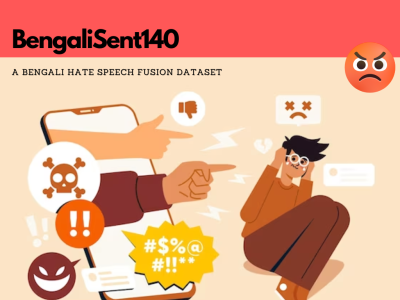
- Citation Author(s):
-
Akif Islam (Department of CSE, University of Rajshahi)Farjana Aktar (Department of CSE, University of Rajshahi)Sujan Kumar Roy (Department of CSE, University of Rajshahi)
- Submitted by:
- Akif Islam
- Last updated:
- DOI:
- 10.21227/0bsx-z948
- Data Format:
- Links:
-
- Categories:
- Keywords:
Abstract
In today's world of online communication and digital media, hate speech has become an alarming problem worldwide. With the advancement of the internet, while people enjoy numerous benefits, there's also a dark side where individuals are subjected to horrendous bullying through hate speech. Tragically, some instances even lead to extreme actions like suicide or self-destructive behavior.
Despite significant research efforts in popular languages like English, German, and French, Bengali lags far behind in these developments. Bengali, being a complex language, faces a scarcity of sufficient data for research in this field. While various methods exist to analyze text online, most predominantly cater to languages like English, overlooking Bengali. However, hate speech in Bengali is a serious and prevalent issue, especially on platforms like Facebook and YouTube. Even television shows sometimes feature comments that are offensive and unsuitable for all audiences. The challenge lies in identifying and combating hate speech in Bengali due to the lack of effective tools in this area, underscoring the need for further research.
A significant hurdle has been the scarcity of Bengali hate speech datasets prior to the creation of this one. This binary dataset comprises approximately 140,000 speeches, among which 68,000 are identified as hateful and 71,000 as non-hateful. It stands as one of the largest repositories for Bengali hate speech online. The dataset was compiled by amalgamating various sources and adjusting labels to denote hate speech presence accurately.
The availability of such data is instrumental in empowering researchers and computer algorithms to develop more effective methods for identifying and curbing hate speech online. This initiative marks a crucial step towards fostering a safer and more compassionate internet environment for all users.
Instructions:
This dataset is primed for binary classification and sentiment analysis of Bengali hate speeches, leveraging a spectrum of deep learning, machine learning, and transfer learning methodologies.
Data Preprocessing: Preprocess the raw text data, including tasks such as tokenization, text normalization, perfect lemmatization and removal of stopwords.
Feature Engineering: Extract relevant features from the text data, such as word embeddings or TF-IDF vectors, to represent the speeches effectively.
Model Selection: Choose appropriate machine learning or deep learning models for binary classification and sentiment analysis tasks, such as Convolutional Neural Networks (CNNs), Recurrent Neural Networks (RNNs), or Transformer-based models.
Model Training: Train the selected models on the preprocessed dataset, adjusting hyperparameters as necessary to optimize performance.
Model Evaluation: Evaluate the trained models using appropriate metrics, such as accuracy, precision, recall, and F1-score, to assess their effectiveness in hate speech detection.
Transfer Learning: Experiment with transfer learning techniques, such as fine-tuning pre-trained language models like BERT or GPT, to further enhance hate speech detection performance.
Deployment: Deploy the trained models to real-world applications, incorporating them into online platforms to detect and mitigate hate speech in Bengali online content effectively.