An annotated dataset of tongue images
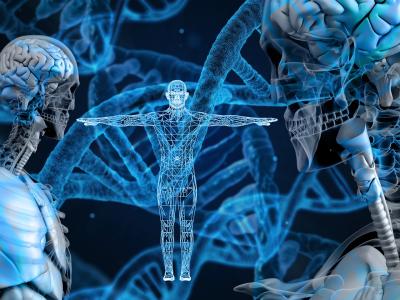
- Citation Author(s):
-
Dan ShiXiao ShiYun Xiong
- Submitted by:
- Chunlei Tang
- Last updated:
- DOI:
- 10.21227/mtt8-q366
- Links:
- Categories:
- Keywords:
Abstract
To develop a non-invasive assessment tool using machine learning in supporting a timely, accurate diagnosis in the elderly, we created an annotated dataset of 668 tongue images collected from hospitalized geriatric patients in a tertiary hospital in Shanghai, China. Images were captured via a light-field camera using CIELAB color space (to simulate human visual perception) and then were manually labeled by a panel of subject matter experts after chart reviewing patients’ clinical information documented in the hospital’s information system.
Instructions:
Subject | Aging |
Specific subject area | Diagnosis – Image and text data analysis Hospitalized geriatric patients are a highly heterogeneous group often with variable diseases and conditions. Physicians, and geriatricians especially, are devoted to seeking non-invasive testing tools to support a timely, accurate diagnosis. Chinese tongue diagnosis, mainly based on the color and texture of the tongue, offers a unique solution. |
Type of data | Free-text document Table Image Each patient has a folder with 1 face image, 1 tongue image, and 2 narrative documents. An additional summary formed by table is provided. |
How data were acquired | We used a patented light-field camera (CN201520303463.5) called the intelligent mirror using CIE L*a*b* color space. Our data acquisition was handled in a standardized way (i.e., ensuring consistent sitting height and placement of the intelligent mirror) as much as possible. |
Data format | The face and tongue images belong to raw data and were taken at 600 pixels per inch (about 42.3 µm per pixel) and saved as a *.jpg with minimum compression (10% compression max). One narrative document is annotated and contains the parameters generated by the intelligent mirror when creating the face and tongue images, and the other contains the annotation results from the expert panel (e.g., vital signs, clinical imaging examination, and laboratory indicators). |
Parameters for data collection | The study was conducted at a Chinese tertiary, comprehensive hospital. We recruited hospitalized subjects (excluding minority groups or other sensitive or disempowered populations) in the Geriatrics Department beginning in January 1, 2019. Images were captured via a light-field camera using CIELAB color space (to simulate the human visual perception) and then were manually labeled by a panel of subject matter experts after chart reviewing patients’ clinical information documented in the hospital’s information system. |
Description of data collection | Data acquisition and image annotation was conducted by subject matter experts including four fully credentialed senior-level physicians (i.e., associate chief physician and above), one resident, and two medical students. One medical student was in charge of data acquisition. The resident consolidated patients’ previous chronic medical history, clinical imaging examination, and laboratory indicators. One physician diagnosed patients’ constitutional types. Another physician gave a final admission diagnosis by considering the patient’s constitution based on both traditional Chinese medicine and Western medicine. Constitutional types are based on TCM analysis and differentiation of pathological conditions in accordance with the eight principal syndromes, namely 八纲辨证, including yin and yang (阴阳), exterior and interior (表里), cold and heat (寒热), and hypofunction and hyperfunction (虚实). All the information from the free-text data labeling was documented digitally by one medical student in Chinese and translated into English. The treatment plan corresponding to the admission diagnosis was reviewed and annotated by the remaining two physicians. A total of 12 items must be merged into an annotated document, including various indices related to tongue diagnosis, physical or mental factors, clinicians’ observations, and more. To mitigate this, we used a previously designed algorithm to generate templates automatically. Under the K-means paradigm, our previously designed algorithm (1) embedded each annotated document into a vector representation for the first 200 patients, (2) partitioned those vectors into several (e.g., K=10) clusters, and (3) designated each cluster representative as a prototype template, or a vector of real annotated document closest to the centroid. For the remaining 468 patients, we used the specified prototype template to assist with the annotation. |
Data source location | Shanghai, CHN Cambridge, MA, USA |
Dear authors,
Thank you so much for making this dataset available to the community which can enable research on this very attractive and high potential area that is integrative medicine (combining both chinese and western medicine).
Best regards
Hugo Ferreira
Dear Authors,
Thanks for this interesting paper and for sharing the dataset.
Best regards,
Narges Manouchehri
In reply to Hello, by Narges Manouchehri
Will share but now is on preparing.
HI
where can we access the full dataset
In reply to Hi, there - we are working on by Chunlei Tang
Hi, we at IIT Delhi nare working on Tongue biometrics. I will be highly thankfull if you could share the data with me on anz208484@iitd.ac.In.Thanks
Hi, we at IIIT Gwalior are working on tongue image analysis. I would be very grateful if you could share the dataset with me at imt_2020056@iiitm.ac.in.
Thanks!