Quality of Service 5G
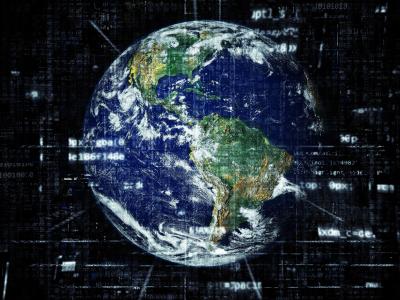
- Citation Author(s):
- Submitted by:
- Erdenebayar Lamjav
- Last updated:
- DOI:
- 10.21227/rzrj-3f59
- Data Format:
Abstract
A rapid growth of wireless communication networks, particularly in 5G Non-Standalone (NSA) deployments, has necessitated advanced multiple access techniques to enhance spectral efficiency, interference management, and energy optimization [1-3]. Rate-Splitting Multiple Access (RSMA) has arisen as a strong candidate to replace conventional Non-Orthogonal Multiple Access (NOMA) by efficiently splitting user data into common and private components. [1-2]. However, determining the optimal rate-splitting ratio and power allocation continues to be a challenging task because of the problem’s non-convex characteristics as well as the requirement for real-time computational efficiency. [2,3].
This study deals with the resource allocation issue in downlink RSMA systems by proposing a joint optimization framework for the rate-splitting ratio and power allocation. The objective function maximizes energy efficiency while ensuring user fairness, minimum SINR requirements, and constrained power budgetsTraditional convex optimization techniques often fail to solve such high-dimensional, non-convex problems efficiently [3]. To overcome this, we propose a Genetic Algorithm (GA)-based approach, which iteratively explores optimal solutions through evolutionary operations, such as selection, crossover, and mutation. Extensive simulations conducted in a 5G NSA environment demonstrate that the proposed GA-based RSMA framework outperforms conventional NOMA and Simulated Annealing (SA)-based resource allocation technique. The results show a 25% improvement in energy efficiency, a 20% enhancement in spectral efficiency, and a 30% reduction in computational time compared to traditional methods. Additionally, Jain’s fairness index remains close to 0.9, ensuring equitable resource distribution among users. The findings of this study indicate that RSMA, coupled with GA-based optimization, offers a scalable and robust solution for next-generation wireless networks. Future research could extend this framework to multi-cell environments and hybrid AI-driven optimization strategies to further enhance the adaptability and efficiency of RSMA in 6G and beyond networks.
Instructions:
None