Stress Detectection via Facial Emotion Recognition using YOLOv11model
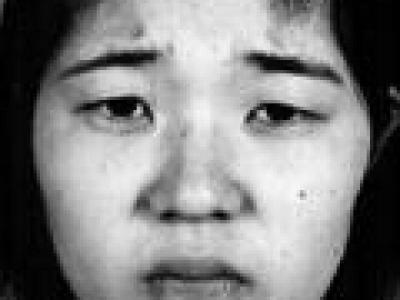
- Citation Author(s):
-
MD. Hasibul Hasan (World University of Bangladesh)
- Submitted by:
- MD. Hasan
- Last updated:
- DOI:
- 10.21227/c9kn-gp07
- Data Format:
- Categories:
- Keywords:
Abstract
In the modern era of fast-paced lifestyles and increasing psychological pressure, real-time stress identification has become a critical component of mental health monitoring systems. This research presents a novel approach to stress detection through facial expression analysis, leveraging Proximal Policy Optimization (PPO)–based Reinforcement Learning and self-attention mechanisms.We introduce a custom-labeled facial expression dataset with seven distinct emotional states-Bursted,Irritated,Anxious,Relaxed,Neutral,Broked,and Shocked to-accurately represent varying levels of psychological stress. A YOLOv11 model is employed for real-time facial region detection, ensuring efficient and high -speed localization in live video streams. The extracted facial features are then processed through a self-attention-enhanced PPO agent trained to classify stress leves based on subtle expression patterns.Experimental result demonstrate the robustness and accuracy of the proposed system in real-time environment, showing significant promise for integration into wearable devices,workplace monitoring systems,and tele-health applications.This work marks a significant step toward intelligent, non-invasive, and continuous mental state monitoring using cutting-edge deep reinforcement learning and real-time computer vision techniques.
Instructions:
To use the dataset you need to download it first into your local machine or if you are using Google Colab then you need to upload it to your Google drivr then use python to integrate it into your model. It uses YOLOv11 Model to detect Facial Emotion.